How to Responsibly Clean, Analyse and use Data
Let’s have a look at stage 4 of responsible data management according to our guiding framework. This stage covers aspects such as data quality standards and data anonymisation. It includes steps for dealing with missing data and a tip sheet table for selecting analysis methods.
Data cleaning and analysis is the stage in the data lifecycle during which M&E data is prepared and processed to generate useful insights. This is an important stage that may require certain expertise such as a data analyst, researcher or even a data scientist if you are working with more advanced approaches.
Analysing quantitative and qualitative datasets should start with a transparent analysis plan which includes steps for cleaning raw data. Raw data often includes errors or consistencies that need to be checked and adjusted during the data cleaning process. Omission of this step could result in inaccurate conclusions being drawn.
M&E practitioners should follow clear guidelines when cleaning their datasets. Guidelines should be agreed upon at organisational level in the form of Standard Operating Procedures and other procedures.
Guidelines for cleaning and analysing M&E data:
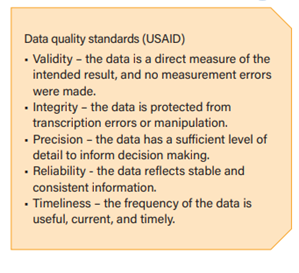
Data cleaning involves addressing incorrect, corrupt and incorrectly formatted data, and removing duplicate entries or incomplete data within a dataset. This process may be onerous if it requires a significant number of data queries to be handled before the actual analysis can take place.
Data quality standards should (ideally) be established at the beginning of any M&E planning process. This is the framework that will guide data quality assurance throughout M&E processes and will also highlight specific steps to be taken for data cleaning after data has been collected.
Many errors in datasets can be avoided by using digital survey tools instead of traditional paper-based surveys. Such tools allow survey designers to incorporate features such as mandatory answers, skip logics, ranges, and to impose limits on the number of surveys submitted
Here are some additional tips:
- Establish clear processes for data cleaning and data quality, validity and integrity
- It is important to systematically organise and document how the data cleaning process will be conducted.
- As the data collected may include personal details of respondents, unique data identification numbers should be assigned for each respondent prior to the analysis phase to ensure the anonymity of respondents.
- When data is collected from a small number of respondents and variables could allow for respondents to be identified, other measures should be taken such as aggregation of results to a level which prevents individual identification.
- The data being cleaned and analysed at this point should be restricted to what is needed for the intended M&E purpose.
- In the analyses of data, protection is paramount, and data should routinely be presented at aggregate level to prevent identification of respondents.
- Devise a plan for dealing with missing values, duplicates, or incorrect values
2. Create and use clean data
A few additional steps must be taken to prepare data for analysis and use, including:
- It is important to first identify the outliers and to consider creating one dataset that includes the outliers and another that does not to assist with providing more relevant analysis.
- Datasets must be checked to determine whether missing data is a true missing field or if it represents a zero or null response.
- It might seem more appropriate to drop entries with missing data. However, this may affect the representativeness of your sample, so the option must be considered carefully
3. Select the appropriate method of data analysis
- Data analysis is the process of seeking patterns in data. This includes quantitative (numeric) and/or qualitative (text, images) data.
- Data analysis fields can be vast with many levels of complexity.
See tip sheet 6 for overview of data analysis and use
4. Other considerations for responsible data cleaning and analysis
Responsible data analysis might be overlooked if we assume that it is sufficient to focus on ethics during the data collection only. Remember that considering ethics at the collection stage does not ensure that data is managed ethically during the entire data lifecycle. It’s critical to also ensure that the data aggregations in your analysis are not exclusionary or prejudiced.
Data patterns alone cannot inform a decision. They must be stress-tested against contextual and other factors. Some questions to ask include:
- Is the trend you identified applicable in all contexts?
- How far can the trend be extrapolated?
- Have you completed sufficient quality checks and balances to ensure that your analysis is sound?
- Have you included representative stakeholders in analysis and interpretation?
- Have you accounted for the sample size and power in the analysis and interpretation of your data?
See tip sheet 6 for selecting analysis methods and uses
Stay tuned to the next stage, Responsible Open Data and Data Sharing as we unpack more on the practical responsible data management tips for M&E practitioners.
See our previous posts on : Responsible Data Management for M&E: Stage 1 – Design and Planning , How to responsibly collect or acquire data for M&E and How to Responsibly Transmit and Store M&E Data to keep up with the discussion, or learn more from the report.
-
Pingback: FAQ: Frequently Asked Questions about Tech & Data | The Engine Room
-
Pingback: FAQ: Preguntas frecuentes sobre tecnología y datos | The Engine Room
-
Pingback: FAQ: perguntas frequentes sobre tecnologia e dados | The Engine Room
-
Pingback: FAQ: Questions fréquemment posées sur les technologies et les données | The Engine Room
-
Pingback: FAQ: Frequently Asked Questions about Tech & Data - The Engine Room