The GBV and VAC research spaces are changing. Are we paying attention?
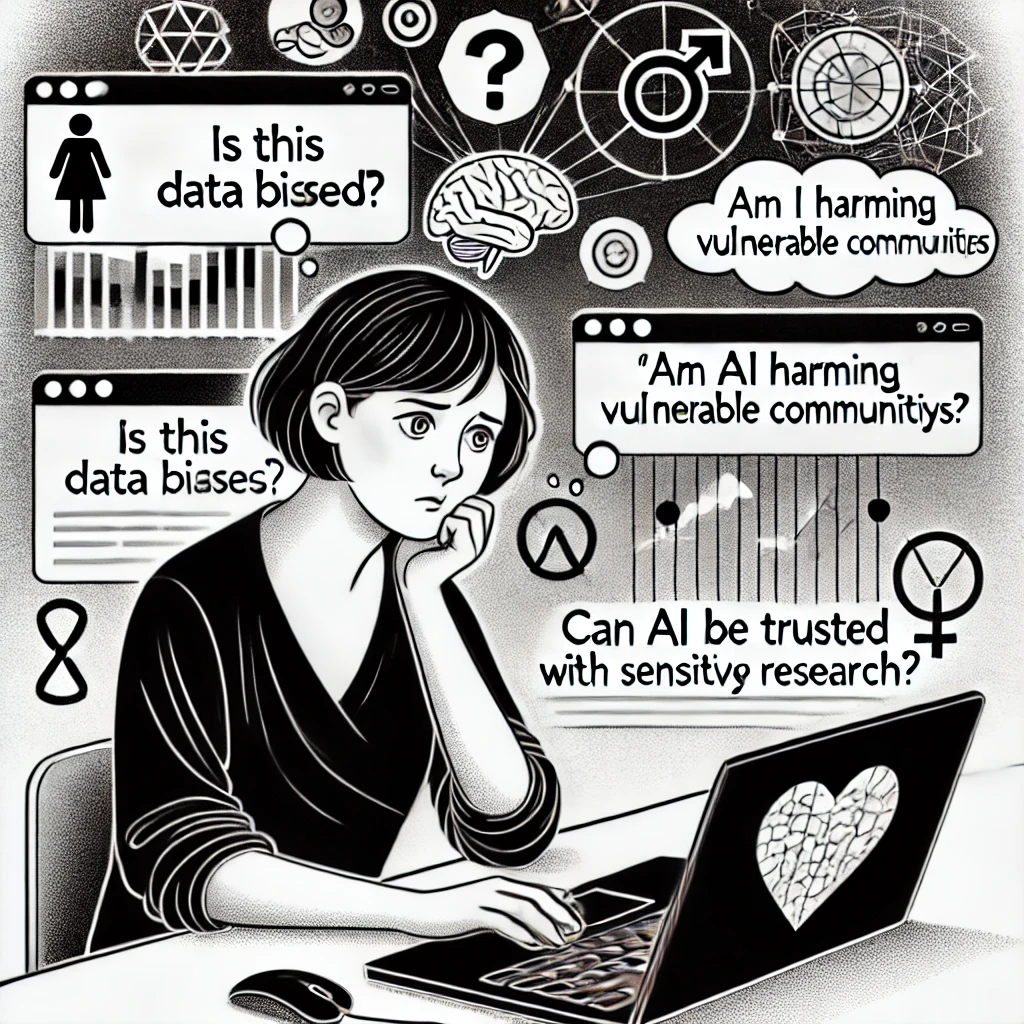
Our November 14th Tech Salon asked whether it’s safe to use AI to conduct research on Gender Based Violence (GBV) and Violence against Children (VAC).
Tesmer Atsbeha, Senior Program Officer, Wellspring Philanthropic Fund; and Diane Chang, Safety Product Manager, Cohere were our lead discussants, and we also heard from a lively group of participants from a range of disciplines and organizations.
Questions we wanted to address at this Salon were:
- How is AI contributing to GBV and VAC? How can it be used to address them?
- Where can AI be used for research on GBV and VAC — from simple, free tools to more advanced data science methods?
- What are the main concerns about using AI in both simple and more advanced ways for research on GBV and VAC?
- Which guardrails do we need to put in place if we are using AI for research in these sensitive areas?
- What questions do researchers have about the ethics and practicalities of using AI tools for research?
Below is a summary of our discussions.
Starting from a power lens
In framing the conversation, our first discussant reminded us that communication technology shifts power, citing the printing press. It’s important to consider AI, especially Generative AI (GenAI), within a power analysis to identify its role and effects in various realms, including research on GBV and VAC.
GenAI could play a role in addressing power dynamics in the field of research. “Just to get to a research conference, to be allowed in the space, to be heard, you need to overcome huge barriers. Can GenAI reduce or lower those barriers? Can it support decolonization and more equity in systems of research?” they asked. “How can GenAI support those who are doing practical, grassroots research on GBV? Not academic research, but folks who are interviewing survivors and who want to be able to use tools to analyze that data and make it more useful for grassroots programs?”
Systemic gaslighting about tech-facilitated GBV (TF-GBV)
GBV research, which was born out of feminist movements, has only been around for about 20 years. Initially, this research was needed to prove the mere existence of GBV. “Fast forward to today, and people still hold that trauma of not being seen or heard. And now we have a new frontier — tech-facilitated GBV — and its connection with offline violence. But AI-facilitated violence is not being seen, recognized, and validated as violence.“
We don’t have laws to protect women and children from deep fakes, said one person whose organization works with survivors of TF-GBV. “There is systemic gaslighting. Local and international law enforcement still don’t believe it’s real, they don’t believe it’s violence.”
Gaps and tension in the GBV research space
Salon participants spoke about the need for more access to aggregate knowledge. While there is a lot of GBV research out there, and there is some agreement on prevalence, we are struggling with TF-GBV. There is no overarching stance on it. We don’t have data consolidated into one place to inform lawmakers or to identify prevention tools that work.
There is also tension. TF-GBV work is in competition with other types of GBV work. Some feel that TF-GBV is getting too much attention and taking away funding from service provision for other types of GBV. This needs to be addressed with funders. It would be helpful to bring what we know into one place. The wider field needs to connect around this issue, noted one person.
AI-related GBV and VAC concerns
Most AI Safety frameworks do not have any type of gender lens. Anthropic, for example, is pitching itself as the company that focuses on safety. “They have an AI Safety Level (ASL) categorization. So, say, if an AI gets to a level 3, then it needs to be shut down. But what elements are being used to categorize safety? We’re seeing how AI is intersecting with GBV. How do we plug gender into the conversations about AI Safety?” Industry guardrails related to AI Safety are wrongly focused on AI sentience, as one person noted. They don’t address current ethical issues and real-world harms that are happening right now, today. [Read more on the difference between the AI Safety and the AI Ethics camps here].
Another challenge is that conversations around AI ethics and safeguarding are happening in boardrooms, not schoolrooms, as another person put it.”ChatGPT is something that 10-year-olds are using. They know how to ask Alexa for things. A typical GenAlpha child doesn’t recognize a separation between on- and offline worlds. They are asking ChatGPT for help with homework and not understanding that it could also open them up to GBV or other risks.”
AI to support research on GBV and VAC?
A common observation about AI use in the non-profit sector has been that people are torn between fear and curiosity. They tend to want to know what AI can be used for in a practical sense and to better understand the concrete risks, how to mitigate them, and where we might need to challenge our assumptions. The MERL Tech Initiative (MTI) is developing guidance on this topic for the Sexual Violence Research Institute (SVRI). Early conversations with researchers and practitioners working in public health, tech and GBV, revealed that many researchers are a bit stuck: “I don’t know what I don’t know, that’s the problem.”
Key questions researchers are asking include:
- How can we protect the data of survivors?
- Can we mitigate gender bias when using AI tools, or will it reproduce patriarchal norms?
- Do we need to disclose AI use? Will we be penalized for using AI?
- Will the use of AI re-traumatize victims?
- Does the use of AI dehumanize the research process? Does it dehumanize us as researchers?
- Can we or should we use synthetic data?
Based on the conversation at the Salon, people are curious about AI but its use for research is limited. If people were using it at all, it was in the early phases of a research process, for example, brainstorming, searching for information and ideas, and getting help bringing in different perspectives. “I use it as a bouncing board – what else is out there, what haven’t we thought about, how can I interrogate the topic? How are other people describing a particular thing? How is it perceived in other spaces?” One person described using it as a way to understand different perspectives on GBV. “I would not know how another country thinks about GBV, so I ask the chatbot. Then I can go back and search and verify sources.”
Apprehension is still preventing the use of AI. “Fear has created a barrier. It’s seen as a risky tool.” This means that people are nervous about trying it out. “We should be playing around with it and interrogating what we feed it and get out of it. Practicing with tech is really important — using it more to fear it less. How many researchers know the available products and are using them?” asked one person. Private sector companies tend to make space for creative initiatives, but non-profits don’t generally have the time, resources, and capacity for this, so we fall behind on our use of new tech.
Another person said that for them, the Salon was sparking new ideas about the use of GenAI for research. “Maybe it’s not about using it for peer-reviewed or academic research. Maybe it’s something we can use for more programmatic research or for design research. But just like any tool, it requires trauma-informed and feminist ways of working. It requires us to resist urgency.“
Others agreed on the feeling of urgency, noting that they’re hearing both “We need to use it now!” as well as “No! We can’t use it at all!” This led to a suggestion that we learn and share in communities of practice, and that we should follow a principle of “working out loud” and develop community agreements around how we practice using AI.
AI as ‘augmentative tech’ for humans
If we think about Large Language Models (LLMs) and AI as augmentative tech, said one person, we can view them as something that facilitates human work. But we need to always have a ‘human in the loop’. An example of how to reduce bias in models is Retrieval Augmented Generation (RAG). “You take the model and run it on your own organization’s data and documents. You can restrict it to your own data. You can have it give you citations, so you know where the outputs come from and how it’s deciding. The tech is improving and that for now is a best practice.”
Humans should still always check the work that the tech produces. It’s also important to understand how effective the model really is for the problem at hand. Different kinds of AI are good at different things. Some work well for classification, e.g., sorting and categorizing. But it’s critical to ask questions about accuracy, one person noted. “Companies will claim they are doing something, but you need to ask what the false positive and false negative rates are. Is it equally effective across languages, cultures, and other subcategories that are important to you? If it’s a predictive model, one that looks at the past and predicts the future, how confident is the mathematical model at building the prediction?”
Another big issue is misconceptions about how bias can be introduced. “Garbage in, garbage out is only one part of the bias issue.” There are many different types of bias found in the underlying models and the applications that are built on top of them. (See this article for more detail). This can include historical bias, labeling bias, semantic bias, representation bias, and others as noted in this paper.
In addition, this Salon participant advised, it’s critical to understand how your data is being used. “If your organization is getting an organizational account, you should be able to choose whether to opt in or opt out of company model training.” When using these tools individually, it’s also possible to opt in or out of data collection and decide whether your data will be used to train AI models. This is often highly confusing, however, for consumers. Companies don’t tend to explain clearly what they mean and what happens with your data when you use an AI tool or add AI to your existing applications.
Funders need to fund differently
Most of the conversation about AI is focused on efficiency and Tech for Good, said one person. “It’s not at all focused on what’s really happening on the ground. How do we get funding for things outside of ‘capital R’ research? Where do we get funding to brainstorm and explore how these tools can be useful for us? Unrestricted funding please!“
Salon participants lamented that funding from tech companies runs on unrealistic timelines that don’t allow for sufficient work on safeguarding and harm prevention. If using AI in humanitarian settings, for example, “being safe is so important! We’ve lost funding because tech funders wanted everything done in 6 months.” There is pressure to launch and scale too quickly, without proper safeguards and risk mitigations.
Shifts in funders and funding models are something to be aware of, said another person. There’s now much less interest in long-standing funding and in building and supporting institutions. “Newer funders and those who have made billions in tech are less interested in funding tech for good. They are pouring their funding into Climate. And they are working with different, shorter-term funding models.” This shapes how civil society and non-profits are being resourced, which is affecting the availability of GBV-specific funding. “We need to integrate GBV research across other sectors instead. Capacity building for tech is in the same boat. We need to integrate these into other thematic areas.”
The whole space is changing — are we paying attention?
While some Salon participants felt that a human filter is absolutely necessary in GBV and VAC work, others pointed out that AI Agents are already being used to interview people about their experiences of violence. This will likely become common in law enforcement and other spaces. Not to mention, while many of us would not want to be interviewed by a bot, some people prefer to engage this way.
One person said that “there are already solutions that are as market-palatable as a real person. Is a bot that’s well trained better than a human?” They suggested that it will be difficult to justify humans doing this work on morals alone if the economic argument is strong. “You can’t moralize people into a good choice. They want something simpler and cheaper. We need to acknowledge that these things are getting built and the easy thing will win out…”
Another big shift is that the nature and place of GBV disclosure has changed among younger people. When we talk about research on GBV and VAC, we are still thinking of disclosure and reporting happening through official channels. One person brought up the fact that young people are disclosing abuse online, for example on TikTok. This becomes their online brand, and they are building community around it, in the open.
“When disclosure happens outside the reporting framework, then the research or body of content that is used to track and trace online experiences of GBV is in the public sphere, not in a report to police. The data is not sitting in a traditional GBV reporting space. How do we find these stories and experiences and keep track of what is happening at a real level?” Traditional research methods are no longer sufficient. How do you create safer spaces for disclosure and support in those online environments? This is how people are building community and it’s not necessarily bad or risky. But how do we research this ethically?
What can we do?
Rather than fearing AI in research on GBV and VAC, what should we be doing? Some suggestions, recommendations, and resources included:
- Use safer tools such as an enterprise version of Copilot
- Develop internal tools that have better data protection (e.g., UNICEF is creating UNIbot)
- Create and use tools that are a fit for specific regions and languages so that information doesn’t get lost, misinterpreted, or misused in the translation process
- Identify solid GBV Research tools, techniques, and platforms that are safe for the sector to use
- Identify and share standards for the use of AI for GBV and VAC research
- Create practical, actionable orientation that emanates from actual practical experiences (for example, update the Safer Chatbots Implementation Guide to include guidance on Generative AI) rather than long prescriptive documents
- Learn from what healthcare is doing: run a pilot, operationalize the metrics and frameworks and address bias and fairness; train and evaluate the AI’s performance and outputs using specific metrics
- Create frameworks and safe spaces for practicing and testing GenAI
- Openly share what does/did and didn’t work in Communities of Practice (CoPs) such as the SVRI’s TF-GBV CoP and MTI’s Natural Language Processing CoP (NLP-CoP).
Technology Salons run under Chatham House Rule, so no attribution has been made in this post. If you’d like to join us for a Salon, sign up here. If you’d like to suggest a topic please get in touch!
This Salon was sponsored by the Sexual Violence Research Institute. We are looking for sponsorship to help cover the costs of preparing and hosting salons – please contact Linda if you would like to discuss financial support for Tech Salon.