AI for Good Partnerships – Old Challenges, New Tech
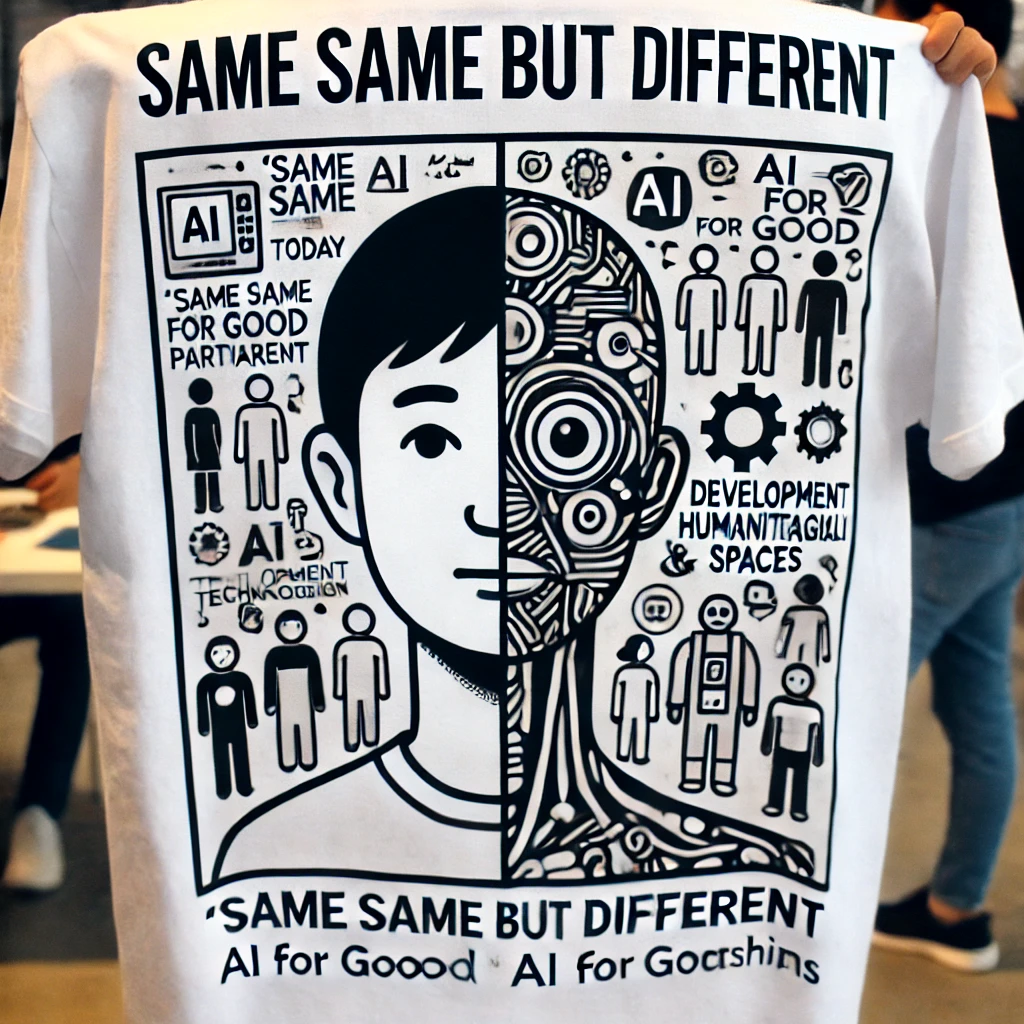
Development and humanitarian agencies are in a strategic position to broker relationships around artificial intelligence (AI) solutions. They often have access to desirable data about un- or underrepresented communities, and they can facilitate the entry of tech and data companies into frontier markets. Without careful scrutiny and purposeful partnering, however, social sector organizations can serve as a gateway for AI partnerships that bring little benefit for communities, work against localization and decolonization efforts, and provide channels for monopolization of national, global, and/or INGO markets.
Concerns about ‘AI for Good’ partnerships include known issues such as extraction of value from data without return of benefits to data holders, experimentation on vulnerable people and groups with untested technologies, and the assertion of Eurocentric values and culture. The GenAI gold rush brings new, unknown unknowns as well. And the narrative in some spaces that “we have a moral imperative to use AI to alleviate human suffering” is problematic.
Bearing all this in mind, our June 10 Tech Salon looked into the question: Are ‘AI for Good’ Partnerships Really Good? with four expert lead discussants (listed below) and some 40 others who are navigating this shifting space:
- Ania Calderon, Managing Director, Strategy and Engagement, Data and Society
- Marla Shaivitz, Director of Digital Strategy at Johns Hopkins Center for Communication Programs
- Hadley Solomon, Global Lead, Ethics and Evidence at Save the Children and Chair, AI Ethics and Risk
- Sean McDonald, Partner at Digital Public and Senior Fellow at the Center for International Governance Innovation
A summary of key points from our discussion is below.
Same same, but different
The discussion opened with an acknowledgement that while AI for Good partnerships present new challenges, they are essentially a continuation of historical ‘Tech for Good’ trajectories. ICT4D, big data for good, data science for social good, and AI for good have all followed similar playbooks. There is a robust evidence base that we can return to for guidance in this new era of Tech for Good.
One core issue with ‘for Good’ partnerships, as noted by one of our lead discussants, is that “we consistently see tech solutions being built for non-tech problems.” These solutions are often designed with no interaction with the people whose problems they are meant to solve, and they are based on incomplete understandings of how social change happens. Rather than solving societal issues, AI can exacerbate existing problems and create new ones.
‘AI for Good’ partnerships may “give by taking away” or essentially become “non-humanitarian humanitarianism.” These initiatives may serve as “a strategy to legitimize profit-oriented practices that serve company and corporate interests. They enable continued use and expansion of AI tools and approaches, often replicating colonialism, tech colonialist, hierarchical, and extractive mindsets.”
Non profit organizations are facing pressure from all sides to adopt AI solutions
As we discussed in our March Salon session, development and humanitarian organizations (and other social sector entities) experience heavy pressure from tech companies, boards, funders and internal Tech for Good teams to adopt AI. Vendors use ‘Trojan horse approaches,’ according to one Salon participant. “They pitch you something, but if you read the fine print, you see that you’ll be letting them put bots into your systems so that they can scrape all your data. They knock on the door. We say no, you can’t have our data. Then they come in from another side with a demo, hoping to get into the enterprise system….”
Funders are also driving the pressure through RFPs that seek quick, scalable AI products with a 12-month turnaround or less. Boards and senior leaders have the impression that funders want this and that “if we don’t do it someone else will.” There is a fear of falling behind, despite knowledge that rapidly deployed AI is not a wise way to invest resources. The short time frame leaves little space for dialogue or adjustments at the early stages. “Safety and community relevance are addressed when the design is far down the road, and by then everyone is too invested to pull the plug or significantly change the product.”
Another big concern, as one discussant reminded us, is that organization leadership may be unaware that many country governments have a back door into this data. To get a license to operate, companies will often negotiate agreements with host country governments that allow domestic security services to access their data.
Weaponized ambiguity
Salon participants expressed concern about ‘AI for Good’ initiatives being parachuted into places with weak regulation and no guardrails regarding intellectual property and data security. They are also worried about the number of requests their organizations get to access their constituents’ data. “AI companies are running out of data from the Internet – they sucked it all out, chewed it up and digested it. So they are looking outside of the US. I have heard Africa referred to as a Data Farm.” Non profit organizations can serve as a gateway to that data.
A big question is “what excatly do they want our data for?” One thought was that AI companies are repeating past behaviors of social media companies. “Facebook’s Free Basics was all about getting data. They are slipping in popularity with Global North organizations, so they are vacuuming up data from the Global South. But if people in the Global South are not high paying customers, it will be hard to sell them their data back in the form of a product. What do we think the goal and the end product are here?” asked one person. Speculation was that it’s not the data, it’s the models that companies can build and sell, for example to governments.
However, another participant suggested that the product is the sale of computational infrastructure. AI companies can sell computation at scale if they can say they have more parameters in their model. “We keep criticizing everyone for being distracted by shiny objects, but we are looking at AI tools as shiny objects! Meanwhile AI companies are mining us for use cases. The real thing to think about is dependence on the computational infrastructure. There is value in high volume computation. A lot of what we’re talking about is how to create pipelines for revenue.”
This ‘weaponized ambiguity’ — about what AI is, what the data is for, what the use cases are, why they want our data — is being used against us, leaving us wondering what the game is.
8 things we can we do to encourage better AI
While the discussion was wide-ranging, we closed out by suggesting some ways we can work collectively to address the issues raised.
- Individuals should get involved wherever they have agency. Most development and humanitarian professionals ignore local politics because we are focusing on national and global issues, but we need to look for ways to engage locally. One person suggested that regulated professions (such as the medical field or the legal field) can accumulate standards of practice that have real potential to shape the landscape. So regulated professionals should not shy away from setting standards and practices for their industries and fields.
- Funders should be investing in AI governance. They should be seeking out creative examples of participatory AI and AI governance rather than funding more tools. They should be asking that certain assessments be done on tools and that AI / data governance are in place (and funding that piece of the process). They should also be funding grants that go beyond AI apps to consider participatory design, implementation, and sustainability of AI-powered products and processes.
- More algorithmic impact assessments. These are being integrated into regulation in the US and the EU. There are tools that center impacted communities along the whole process. These should include better ways for assessing tradeoffs. More accurate, less biased, more privacy preserving systems will likely demand more energy and will be less inclusive because they are more expensive, which leads to more concentration of power. We need ways to assess the trade-offs across these aspects.
- Set internal guidance on our own use of AI. Is it OK to use AI to drive ‘for good’ messages? What are the parameters for ethical use of AI when done by local organizations with ‘positive’ messages or in political campaigns for pro-democracy candidates? When can we plug our constituents’ data into AI? A domestic nonprofit AI use policy would be useful, including a list of questions to ask vendors before working with them. The American Library Association, for example, has a task force that is looking into AI guidelines and recommendations for libraries to guide use of their data and other resources.
- Govern AI at multiple levels in various ways. There’s no one size fits all approach to AI governance. What is the equivalent of regulating AI like we regulate transport? At the level of city, state, nation? At the level of roads and highways and interstates? At the level of car manufacturers and emissions and vehicle safety? At the level of seat belts and not driving drunk? At the level of street signs, traffic lights, and road rules? How can we get involved and involve others to shape this governance?
- Work with the arts communities, activists, social movement leaders, libraries, and journalists. Currently tech companies own the narratives about AI. We need to work to offer alternative narratives. Could we bring in cultural and arts organizations to develop events and installations that help the public (and decision makers) better understand AI and AI governance? Could we create speculative futures projects? Could we better bridge the tech, human rights, creatives, and arts communities? Can we better work with journalists and the media?
- Shift power and dependencies. Is there a community ownership model for computation? How can we put more effort into that, or create new models for these related areas and dependencies? What kinds of public participation can we bring into these areas?
- Improve AI and AI governance literacy. This includes educating legislators and decision-makers on the ‘sociotechnical’ decision tree so that they make better decisions about AI use and AI governance. It also includes improving our own internal literacy for grant writing and adopting emerging AI in responsible ways. And it includes funder education on what responsible AI looks like and how to develop funding calls that prioritize safe, ethical, sustainable processes when AI is involved.
Technology Salons run under Chatham House Rule, so no attribution has been made in this post. If you’d like to join us for a Salon, sign up here. If you’d like to suggest a topic please get in touch!
We are looking for sponsorship to help cover the costs of preparing and hosting salons – please contact Linda if you would like to discuss financial support for Tech Salon.
-
Pingback: AI. What Is It Good For? - Art Meets Code
You might also like
-
October 16: Build a ‘no code’ chatbot in under 5 minutes
-
Check out these AI- and tech-focused sessions at the European Evaluation Society Conference in September
-
Learn more about Safe AI for Children and Young People at our October 3 session
-
Push that boundary! Hold that boundary! Exploring multiple paths of inquiry for AI and Evaluation