10 ways to help your organization manage the pain of AI adoption

Our March 11 Technology Salon in New York City welcomed managers and directors from some 25 organizations for a discussion (therapy session?!) on how to manage the pain points of Artificial Intelligence (AI) adoption at development, humanitarian, and other non-profit organizations.
Kick-starting the discussion were Friederike Schüür, UNICEF’s Chief of Data Strategy and Data Governance; Ronen Rapoport, Business Relationship Manager & AI Taskforce co-lead; Conor Flavin, Senior Data Policy Officer at UNHCR; Mala Kumar, Former Director of Tech for Social Good, GitHub; and Brent Phillips, Humanitarian AI Today. Salon participants joined in actively and vocally as well! We were graciously hosted by the Rockefeller Foundation.
Discussion recap:
The AI gold rush
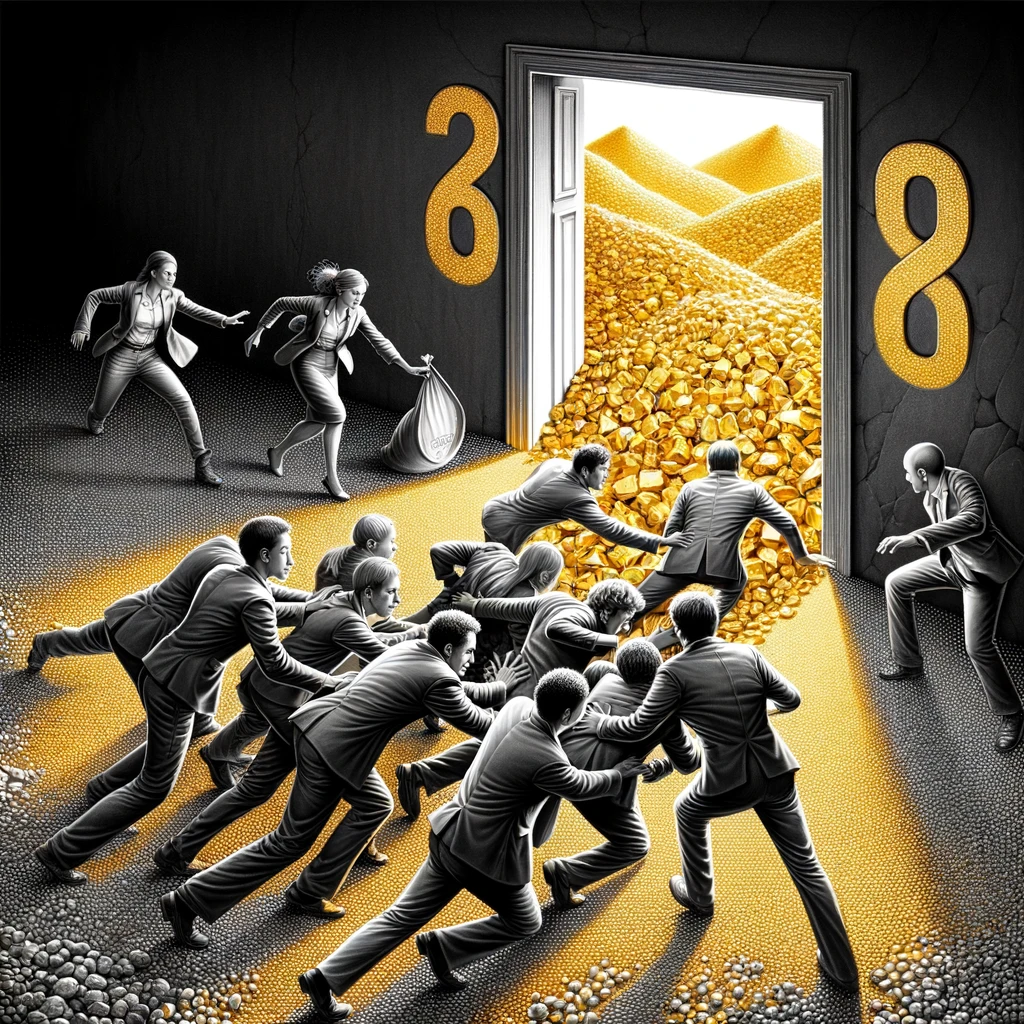
Some Salon participants felt that the emergence of GenAI in November 2023 brought about a watershed moment. A few years ago we saw small scale, disparate AI-enabled projects, but now people are rushing towards AI, fearful of being left behind. “Everyone wants to be the center of gravity on this,” said one discussant. “People want to get started but at the same time they are afraid.”
As one person described it, “We’re all rushing to the goldmine — but we end up blocking the entrance! Innovations, strategy, data governance, data protection, policy teams…. Everyone feels they have an important part to play in this,” said one person, to which another joked: “Some are rushing to find the TNT so that they can blow the whole thing up, and other are scrambling to find the first gold nugget and claim success!”
Others felt that the importance of GenAI depends on your vantage point. “We work in 120 countries. While on the one side we’re pushing sophisticated GenAI, on the other we’re working in places that might not even have a word for ‘data.’” Generally, though, Salon participants felt that something changed with the public launch of ChatGPT. “It’s causing us to question what we are doing, and what we could be doing,” The major breakthroughs in this type of AI happened 8 or 9 years ago, but now “a lot more people are paying attention. Our job is channeling that energy into productive pathways.”
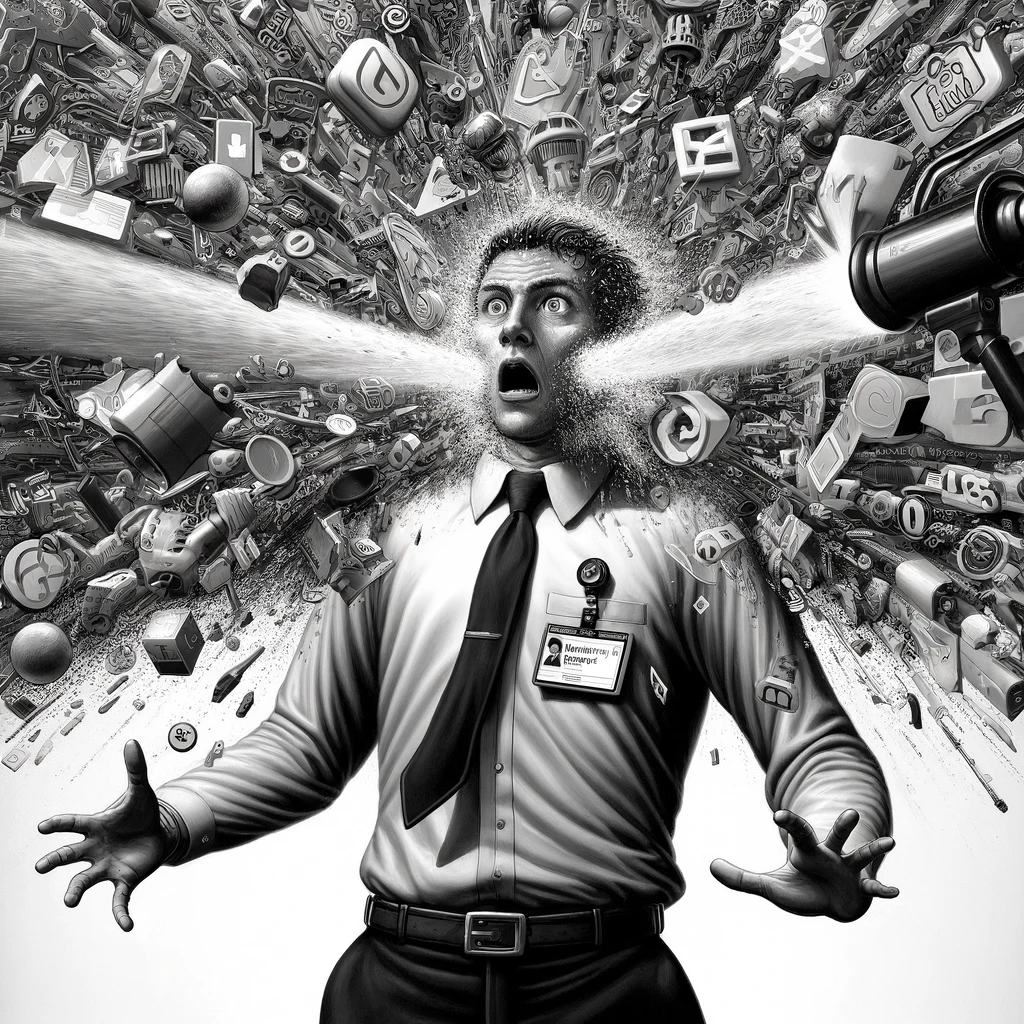
So much noise!
As one person put it, “I spend half of my day fielding questions about AI: ‘What is this all about?’ ‘Can it really write my talking points for me?’” Senior leaders are bombarded with AI all day long. “Microsoft is all over it. Zuckerberg is all over it.” The problem is that NGOs and UN agencies are not defining what they want and need from AI. Rather than letting AI lead, organizations should be identifying what outcomes we want and then looking at whether there are ways that AI can contribute to solving things like conflicts or climate change. “All the noise is motivated by profit, but we have a responsibility and the power to define what we want. In all this noise, the greater question of accountability will come to the fore – accountability on big tech. This is the elephant in the room,” noted one person.
Internal facing AI vs community facing AI
We forget where this tech comes from, said one discussant. “Who is trying to build it? Who was it built for?” The pattern tends to be a big tech breakthrough, a lot of investment goes into the private sector, and then the NGOs come in trying to see how to use it. But we are an insignificant share of the market for these companies. The main way that AI is profitable right now is in Business-to-Business (B2B) automation for giant companies who can save a huge amount of money by being even 1% more efficient. So this is where the investment and experimentation are happening.
Companies are mandated by law to comply with regulations to avoid violating certain rights, but they are not legally mandated to use AI for good, to achieve positive outcomes. So while we might see efforts at legal compliance, we’re not seeing huge investment in proactive use of AI to address the social issues that are part of our non-profit mission.
NGOs should start by integrating AI into internal business processes rather than going straight to programmatic use of AI, suggested one person, because we don’t have the budget or capacity to use AI responsibly in programs. Another person agreed. “Our overhead is restricted by charity watch dogs. We lose our rating on Charity Navigator if we go above a certain percentage. So just 2-3% of our budget goes to cover our entire IT and data strategy.” This means that NGOs are severely handicapped when it comes to safely exploring the use of AI for programs.
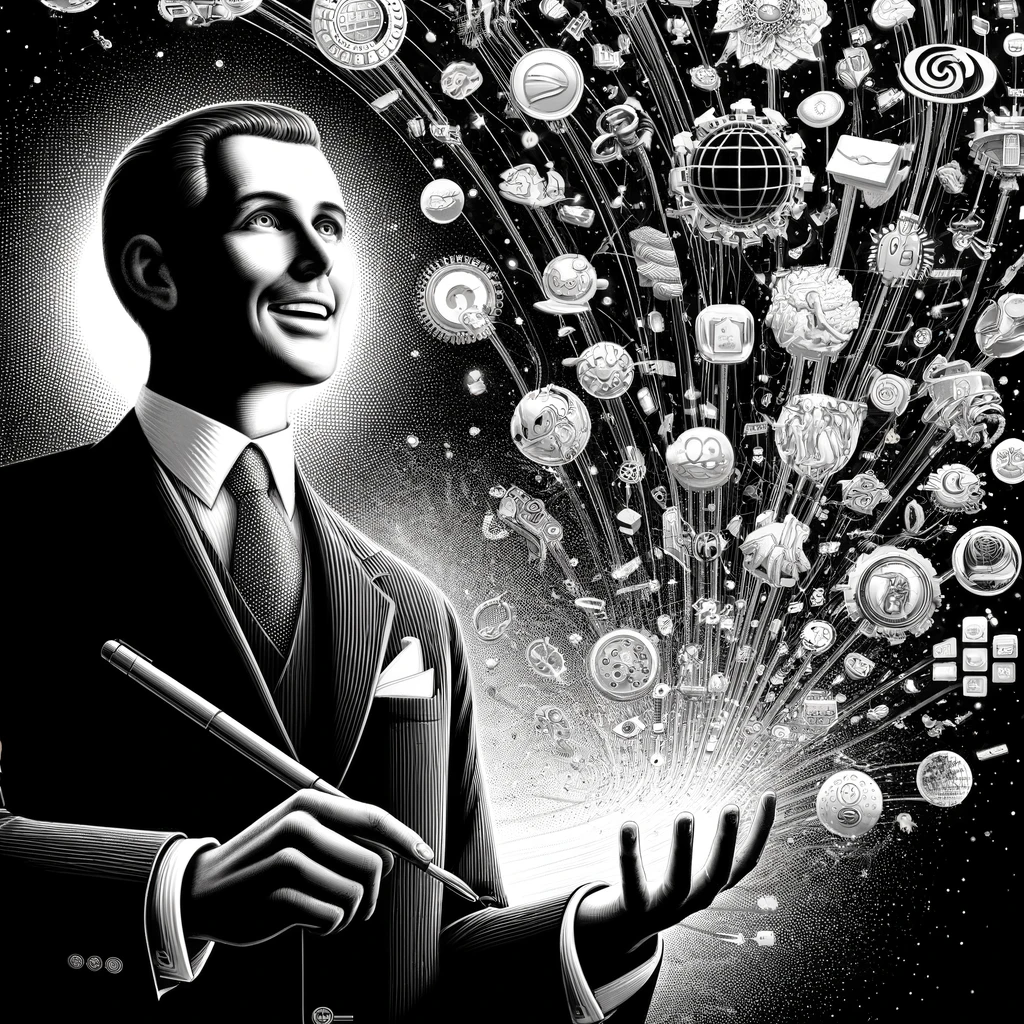
Optimizing for rights is a lot harder than optimizing for profit
“Our work is so complex,” said one person. “We’re unpacking so many layers.” Agencies like the UN are massive and multi-sided with multiple-stakeholders. Large agencies can certainly optimize business functions with AI. “But let’s unleash AI on our colleagues first, not on children. Let’s be aware of the power dynamics. Let’s experiment with people who feel empowered to complain.” Another person agreed “This keeps me awake at night. The notion of a sandbox [for safe experimentation with new tools] is noble when you want to test things on powerful people who work at tech companies and are making half a billion dollars, but that’s not who we work with.”
The tragedy of the demos…
“Every tough discussion I have starts with ‘I just saw a demo.’” chuckled one person. “Then we have to walk it back, ask about what it would cost, what are the parameters and the risks, what infrastructure we’d need to operationalize it.”
“But how do we qualify these demos when they look like magic?” asked another person. Demos tend to be perfectly calibrated with “a golden data set” but don’t perform well at larger scale or with an organization’s own data set. People are often not aware that what they are seeing is a nice visual (a ‘picture prototype’) about a potential application, not a functional tool that is drawing from actual data, code, and a full tech stack (a ‘coded prototype’). “A demo is one thing, but delivering the coded prototype is something else, and our staff don’t know the difference.”
“Our C-Suite and our board are inclined to believe the noise about the promise, and ignore the noise about the threats,” lamented one person. Others agreed: “Someone will walk in and demo something that looks great, but we don’t have the technical capacity to take it further or conduct due diligence on the company or its product to know if it actually IS great.” Facebook’s No Language Left Behind model is one example: “Sure, the computer power is huge, but the model is actually super limited!”
AI literacy is low at most organizations
We don’t even know what questions to ask when we see a demo, said one person, because most of us don’t understand AI. Of the organizations present at the Salon, none had solid due diligence processes in place for vendors and AI tools. One person highlighted that the most important thing is ensuring transparency from the vendor about accuracy and about what a tool is trained on. Yet on top of transparency, organizations also need empowered users who know how to interpret the answers to due diligence questions. These literacy issues are even more of an issue when an organization hasn’t determined how it will approach AI.
10 Recommendations for moving forward
It was not all doom and gloom however. Many are proactively working to find ways to safely steward their organizations forward in this area.
1. Be humble!
If your role involves shepherding AI into your organization, it’s important to know how to have humble conversations with people who don’t understand the technology. “70% of my job is translating and putting this information into bite-sized bullet points,” said one person. “I need to be able to have a conversation about this with people who don’t know how to use Excel. I also need to know how to hire locally and build capacity, and to manage all these layers and partners. It starts with humility and playing the interlocutor role.”
2. Create an AI Working Group or Task Force
This appears to be a first step for most organizations. This can get political “because everyone wants to shine on the new shiny thing.” It’s helpful to bring those voices all into one place, but be aware that determining who “owns” AI in an organization can be a highly political exercise. “Who owns AI” was considered a silly idea for some Salon participants. “All of the elements that sit under AI need owners, so various teams need to be involved.” An AI working group or task force plays an important role in sending clear and consistent messages to staff. Without alignment, different teams will be telling staff and partners different things, creating confusion.
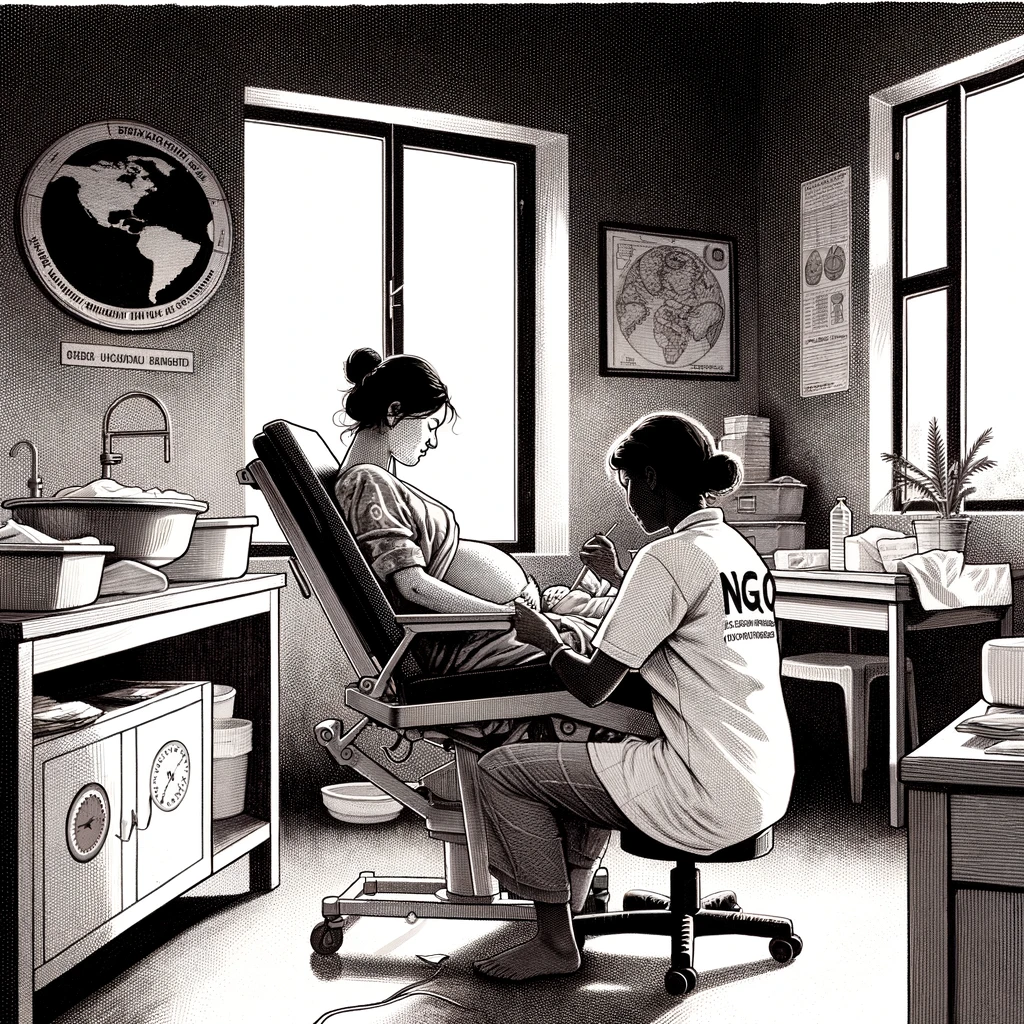
3. Appoint an “AI Doula”
“We have the people who are saying ‘Wait! we need a strategy before we act!’ And then we have the ‘but I just saw a demo!’ people.” A Chief AI Officer or other high level role can help to pull these strands together. This role has to be senior enough to influence decision makers and have a strong voice across the organization. One person described this as a kind of “AI Doula”, who helps safely deliver AI into the organization and reduces risks of a bad delivery.
4. Develop an AI Strategy
Participatory development of an AI strategy can help to get people around the table to discuss, learn, and agree on a way forward.
5. Create guidance on internal use of AI
There are a number of guidelines that organizations may need, including for staff use of commercial AI tools at the individual level; for teams that are building AI internally; for assessing and conducting due diligence on AI vendors and products; for partnering on AI initiatives; for data protection and sharing when AI is used (including how to manage consent); for use of AI during monitoring, evaluation research and learning (MERL); for IT selection, adoption and ongoing management of AI tools at the enterprise level; and so on. These might be separate guidance or integrated into existing guidance and policy. Given the rapid evolution of AI, some organizations are focusing on discrete guidance for now rather than policy.
6. Create a learning environment
When creating guidance or managing people’s excitement around AI, it’s important to strike a balance between hard lines of what you can’t do, and supporting teams to conduct and document safe experimentation. “We’re in an initial phase of something new. It’s like, when my child runs off, I might want to scream ‘no!’ But if he’s just going to fall and bump his head, it’s OK to let him learn by doing. Let’s say ‘no’ to using AI for mental health and GBV. But let’s say ‘yes’ to creating new textbooks with AI. I want to watch as people learn so that we can inform the next project. That’s how we can create a learning organization.”
7. Use the energy surrounding AI to get the basics funded
People don’t generally understand “all the other stuff you need to fund if you want to use AI.” Some organizations hope to use the energy around AI as a catalyst to get budget approved for improving capacity for the areas that need to be in place for using AI: data, cybersecurity, data protection, data literacy and more. “We have 130 offices but we don’t have skilled people who understand data and AI. How do we get funding in the right ways for this?”
8. Collaborate
Some suggested that organizations could work together on some focused areas. Others suggested tapping into open data sets or working with the private sector to create sandboxes for experimentation.
9. Don’t be naive about private sector partnerships
One person advised being very careful about private sector partnerships. “Look for where the team that reached out to you sits in the org chart,” she said. Is it the sales team? The foundation or .org team? The CSR team? Are they just looking for PR? Are they using you to get data and models they can’t otherwise access? Who gets the IP? Are they planning to keep the IP and license it back to you or do they get a license to your data in perpetuity?” Lots of organizations don’t realize that their data is valuable and it’s important to know the answer to these questions before entering into partnerships so that you don’t get take advantage of.
10. Be smart about skilled volunteer offers
Working with skilled volunteers is one way to get data science support in house. These projects should be assessed carefully in terms of sustainability once volunteer support ends. If the skills to keep a project going don’t exist in-house, it might not be a good idea to do the project.
Technology Salons run under Chatham House Rule, so no attribution has been made in this post. If you’d like to join us for a Salon, sign up here. If you’d like to suggest a topic please get in touch!
We are looking for sponsorship to help cover the costs of preparing and hosting salons – please contact Linda if you would like to discuss financial support for Tech Salon.
-
Pingback: An AI Utilization Framework for Evaluators Seeking to Responsibly Incorporate AI into Their Analytics Process by Elizabeth DiLuzio – AEA365
You might also like
-
Join us on May 15th for an AI+Africa Working Group Meeting: Shaping Our Priorities!
-
Event Recap: Tests of Large and Small Language Models on Common Evaluation Tasks
-
Girl Effect Guidelines on Ethical AI-Powered Social & Behaviour Change Chatbots
-
Tech Salon recap: listen more and shift away from Western-centric framing to better address online violence against women and girls