What does participatory design look like in the age of AI?
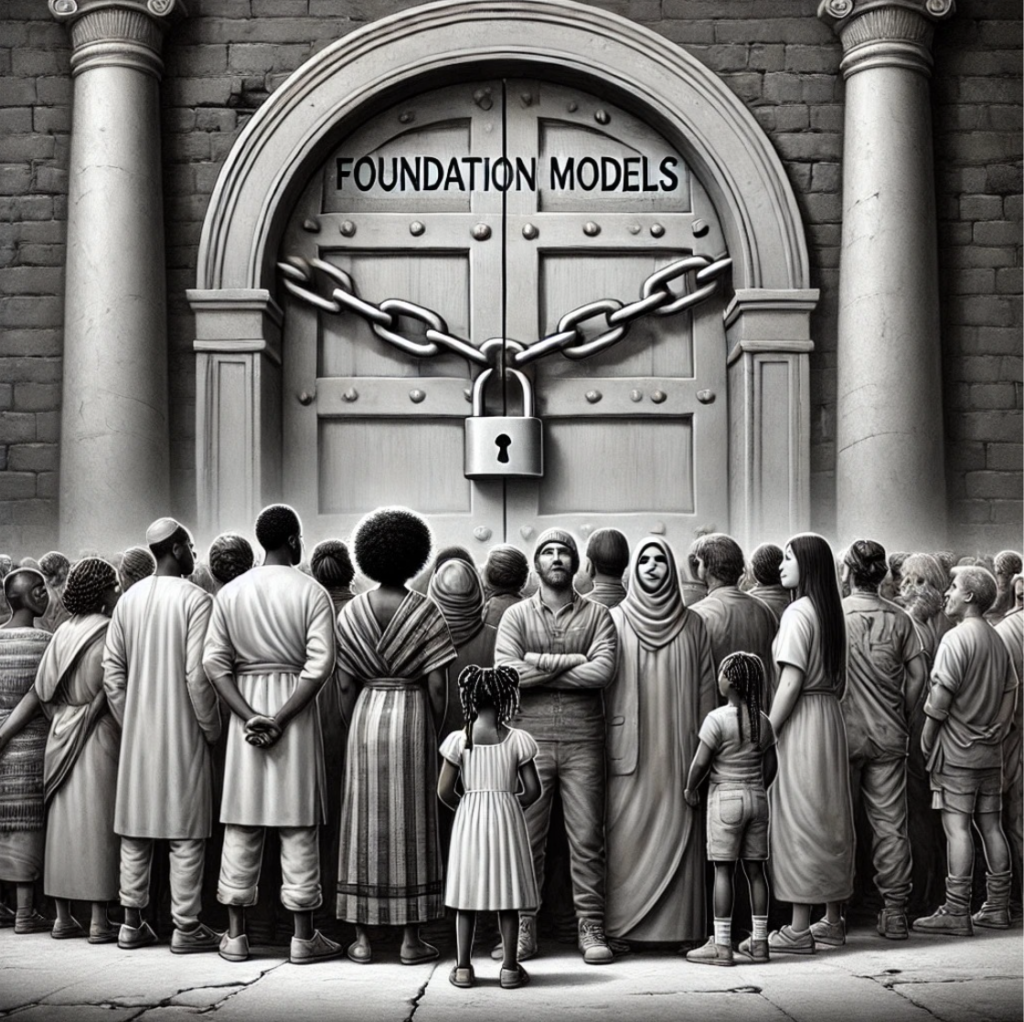
A fascinating new paper argues that foundation models, like those used to power ChatGPT, are actively undermining participatory approaches to social development because of their emphasis on universality and scale at the expense of context-specificity. This is an important issue that those working in the social sector and using/designing tech should be aware of. At the same time, there are many practical ways that GenAI when used “downstream”, might help improve participation.
In this post, I argue that whilst there are core issues with how AI itself is developed which impact participation, organizations and their constituents can also use it to enable more agile, more inclusive participatory activities in development programming. Maintaining momentum with participation and inclusivity is ultimately tech-agnostic and depends on the will of our sector to challenge the top-down status quo.
*
Even though participatory approaches to development have been around since the 1970s, I spent the early part of my ICT4D career in the 2010s trying to convince funders and INGOs that including time and budget to work with community members on designing digital interventions was worthwhile. But the enduringly paternalistic nature of international development along with the reality of tight timelines and budgets meant it was all too common to launch a mobile website, chatbot, or even SMS campaign without ever speaking to a user, let alone setting foot in-country.
Fast forward a few years, and participatory approaches and language — re-packaged as Human Centered Design — have to some extent been mainstreamed: tools are “co-created”, programs leverage “design thinking”, and services aim to be “user centric.” (I should know, my website is stuffed with this jargon!). In theory, what this means is that today, more community members tend to be engaged, in more active ways, at more stages of a programme’s implementation.
This is undoubtedly to be celebrated, although I would argue that as a sector we are still a long way from the next rungs of the participatory ladder, and more often engaged in what Arnstein calls “degrees of tokenism”. So how celebratory, exactly, should we be?
Foundation models are incompatible with participatory principles
Foundation models are AI systems, trained on vast data sets, that can be adapted to a wide range of tasks, for example through specific training or fine-tuning. A recent paper, “Participation in the age of foundation models’, argues that AI systems are by nature incompatible with participatory principles because “downstream use cases and stakeholders are disconnected from the model both conceptually and practically”. Where participatory approaches such a Participatory Action Research stress the importance of context-specificity, foundation models by their nature aim for universality and scale. As such, the authors argue that there is a ‘participatory ceiling’ inherent to foundation models – a point beyond which participatory approaches become untenable or meaningless because of the impossibility of redistributing decision-making and power at this level of operation.
What does this mean in practice? Let’s imagine that we are going to use a model such as ChatGPT to power a chatbot designed for youth in Kenya to get access to reliable sexual health information. A couple of years ago, it would have been possible (though not always feasible) to not just involve, but actively co-design most aspects of the chatbot with end users. This is because with a decision-tree style chatbot, or one using predictive AI, the output of the chatbot and the logic behind its responses are almost entirely controllable by the organization who has set it up. You can sit in a room with users and, with some coaching, come up with labeling rules for a predictive AI model, for example. Similarly, your users can be actively involved in problem formulation, decisions on how their data is handled, and even how to measure the success of the tool in the short and longer terms.
Creators of foundation models have tried to make them more participatory – or at least more inclusive (which often has more to do with commercial viability than as part of a real interest in democratization) – through processes like Reinforcement Learning from Human Feedback (RLHF), community inputs into rule sets and policies, and red teaming. Yet very few of these efforts go beyond ‘inclusion’ to achieve real collaboration, let alone ownership.
The above-mentioned paper raises interesting points, but I’m not sure if there is anything fundamentally different about this state of affairs than, say, our use of Meta’s social platforms, or our reliance on Google for the discoverability of digital development services. Under both paradigms, we are leveraging technology which has been designed with little to no input from the community members we work with and for. Similar to how many non-profits embraced Facebook’s efforts to penetrate markets in LMICs with initiatives like Freebasics a few years ago, we are enabling Big Tech to access Global Majority user data for profit and thus contributing to the entrenchment of tech monopolies. Just like with AI, we often don’t fully understand how tech companies use this data.
So what opportunities do we have to push at this ceiling, other than ongoing efforts in fine tuning or sector specific red-teaming? One idea suggested by the authors was also raised in our Ethics & Governance Working Group discussions: the importance of creating communication channels and accountability mechanisms between domain-specific experts such as ourselves (as experts in global development, or social and behavior change/SBC) and the gatekeepers of foundation models. Whilst some organizations, such as Turn and the International Rescue Committee (IRC), are partnering with OpenAI to support the development of ‘for good’ GenAI tools, there seem to be no avenues (or interest in creating them) for feeding the unique insights and concerns of our sector ‘upwards’, and, theoretically, influencing decision-making that will impact us and our users.
3 big areas where GenAI could increase participation
While we keep agitating for more participatory avenues to influence Foundation Models, at a more tangible level, I still believe GenAI can actually facilitate more participatory work if deployed thoughtfully at all stages of digital program development. I have some ideas on how and would love to hear more examples.
- During program design, it can be used to surface and synthesize existing research and insights relating to community members, to avoid duplication and amplify voices and opinions that have already been solicited by others (though this will only happen if we become more transparent with our outputs and data). KORE Global, for example, have noticed that survey respondents are using GenAI to help craft their responses, for example to translate them from a local language. Whilst this does raise challenges from a data cleaning point of view (in cases where respondents have simply asked an AI assistant to provide them with an ‘acceptable’ answer), it could also be considered a work-aid that makes taking part in surveys that will go on to inform policy or programs more accessible and inclusive. What if, since we’ve observed this behavior, we started to design our surveys to capture the original language and also offer embedded translation so that we could draw in original inputs as well as make it more comfortable for those who need language or translation assistance?
- During design activities, GenAI tools could be used in a variety of ways which increase accessibility and efficiency. For example, non-native language speakers sitting in on Focus Group Discussions might use translation tools to rapidly understand participant inputs, and vice versa, enabling the nuances of answers and questions alike to be better appreciated.
- With the major caveat that the most sophisticated models tend to be behind a paywall (costs would therefore need to be covered by the facilitating organization, in most cases), image, video, and even HTML code can be generated in collaboration with users, offering more high fidelity alternatives to paper prototyping during co-creation – including remotely. Similarly, GenAI powered chatbots themselves can be created using free to access AI assistant builders like Hugging Face or Dimagi’s Open Chat Studio, and tested more rapidly in direct collaboration with users, where previously only role-play activities could enable users to craft and dissect conversations that could be turned into a chatbot experience.
- Finally, AI could also increase participation during MERL activities – an area from which users are often excluded except as sources of impact data. For example, I would like to see more of us using GenAI to generate accessible reports and decision documentation specifically for research participants, as we did at Here I Am, to enable program users to engage with and respond to them, should they wish.
It can sometimes feel like the greatest impact of a programme with a complex theory of change – which may or may not come to fruition – comes from users’ participation in face to face workshops which enable them to engage with new concepts and skills (such as design, or content development). In a similar way, perhaps we can create unintended impact by being more transparent and democratic with our users, with the help of GenAI tools, inching us ever closer up the ladder towards partnership rather than consultation or tokenism.
Ultimately, ‘real’ participation, along the lines laid out by Arnstein and others, has nothing to do with technology and everything to do with our own willingness to decolonize development and decentralize decision-making.
Hi! I’d like to ask you more about how you used GenAI to generate accessible reports and decision documentation specifically for research participants in Here I Am. Is there anything else you can share? We always struggle with this, in particular with young people.
Cheers
Hi Lucia – thanks for your question (and hi to the SDD team, worked with you many moons ago on the Voices 4C Change project!). To answer your question, I would say part of the challenge is not a technical one, it’s a time one – the time we need to commit to in order to do this consistently and properly. It’s also based on the fear (or assumption) that the sometimes obscure or maybe frustrating decision-making processes that go into programme decisions can be hard to convey, so we end up not bothering. What I’ve found is that a little goes a long way – so just setting yourself a guardrail of e.g 1 page, or 2 paragraphs, that roughly summarise next steps, insights or decision making, in very simple language, is sometimes enough. You can use GenAI to help you do this, but bear in mind that feeding proprietary reports (for example) into ChatGPT or similar may not be appropriate. Be experimental about it – try one format, get feedback, and go from there. I hope that helps a little.