Recap: Cutting through the Noise – Finding Meaningful Uses of AI throughout the Evaluation Life Cycle
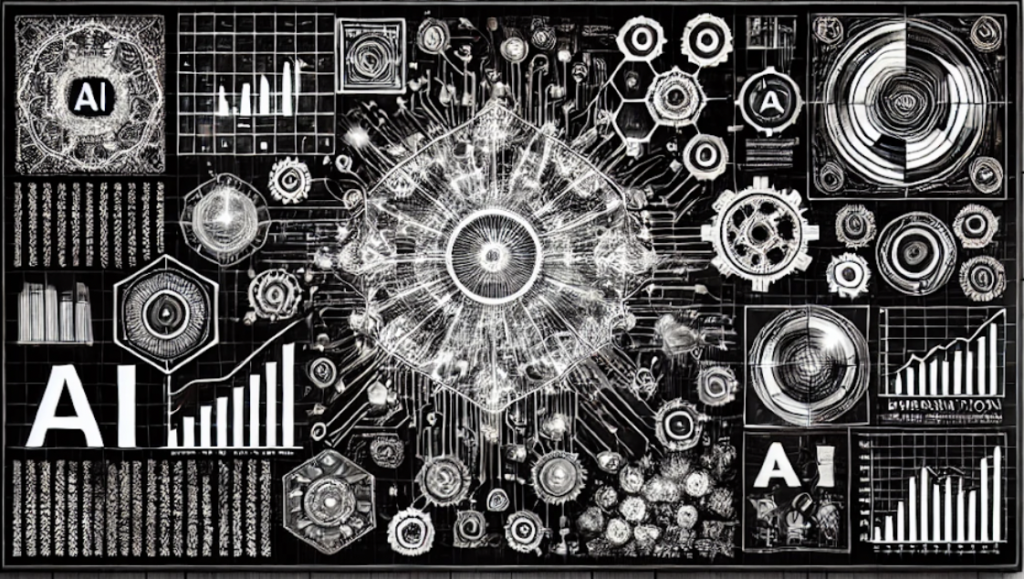
by Brad Krueger and Meghan Adams independent evaluation consultants from Krueger Consulting
On July 25, 2024, we joined the Sandbox Working Group of the Natural Language Processing Community of Practice (NLP-CoP) to share how we are exploring entry points for evaluators just getting started with AI in their work. You can access a recording of our full session here.
At this session, we shared our journey of integrating AI into our evaluation practice and offered practical insights for fellow practitioners.
Feeling left out, not technical enough, and not sure where to get started, we set a goal to cut through the noise of AI in 2024. Following months of learning, experimentation, and now daily use, we shared our experiences and advice on how to get started, both in terms of which tools and which tasks.
Navigating the AI Landscape
With over 5,000 AI tools available, choosing the right one can be overwhelming. We suggest a pragmatic approach: focus on the few large language models (LLMs) that power most tools, such as Open AI, Anthropic, or Llama, etc. We recommend using one of the major, off-the-shelf, turn-key options for daily use, considering factors like existing software ecosystems, cost, core functions, and unique value propositions.
AI Throughout the Evaluation Life Cycle
At the session we outlined numerous ways AI can support evaluators throughout the entire evaluation process:
- Design Phase: AI can assist in learning about topics, summarizing RFPs, outlining proposals, drafting needs assessments, supporting logic model development, performing literature reviews, drafting evaluation questions, and preparing project materials.
- Implementation Phase: Use cases include developing data collection toolkits, creating scripts for focus groups or interviews, planning outreach, suggesting quality checks, assessing project status, and scoring assessments.
- Use Phase: AI can partner to help with data cleaning and review, recoding data, providing language assistance (such as R, excel, or python), developing visualizations, suggesting key insights for different audiences, and tailoring communications.
Best Practices for Using AI in Evaluation
Some valuable tips we’ve found for effectively integrating AI into evaluation work include:
- Building prompts using the POP framework: Persona, Objective, Parameters.
- Letting the AI do the heavy lifting, but breaking complex tasks into manageable steps.
- Providing feedback and requesting revisions as needed.
- Tailoring the final output to your specific needs.
- Don’t get discouraged – there’s a learning curve, but AI is designed to help you along the way.
- Be mindful of privacy concerns, especially when handling sensitive data.
Looking Ahead
We encourage evaluators to dive in and start using AI tools purposefully in their daily work. We believe that while AI is already powerful, it will continue to improve with time. The key is to start the learning process now and stay involved in the evolving field.
We concluded our session by sharing a few studies highlighting the benefits for speed and quality in work for those leveraging AI tools and emphasized how it (AI chatbots) can complement human intelligence but can not replace it.
As the field of evaluation continues to evolve, it’s clear that AI will play an increasingly important role. By embracing these tools responsibly and intentionally, evaluators can enhance their practice, increase efficiency, and ultimately deliver more value to their clients and stakeholders.
Participant Questions
Following the 35 minute presentation, participants shared their questions and insights. A few key ones included:
Question: How and when do you talk to clients about using AI as a tool in your daily work?
Response: We shared that we do disclose use of AI, but clients don’t normally ask which specific tasks are being assisted by AI tools. We do ensure data integrity and privacy is being maintained as described in any contracts and we focus on the quality of deliverables that clients expect from us. Several participants mentioned how different tasks, settings, and contracts demand unique considerations for disclosing use. As an example, we wouldn’t likely disclose re-wording of a sentence, but qualitative coding assistance may warrant disclosure.
Question: How do you balance the time saving AI can provide with the time to review the output for quality and accuracy?
Response: We feel that it depends on the task. For example, drafting a rubric vs completing a needs assessment draft are very different. The rubric can be developed by AI very quickly and saves considerable time, and the human review is quick, a clear time savings. Drafting of a needs assessment is a quick task for AI as well, but given the complexity, amount of data being pulled, and nuance, it will require significantly more time to review. The net time savings may still be there, but probably not as clearly with the previous task. Assessing your use cases for AI tools is important, but, as you are learning and navigating where it fits well, the presenters encouraged experimentation.
We closed the session with a reminder that AI tools can be beneficial even for evaluators without technical expertise and that the media hype shouldn’t distract us from finding use cases that support our work.
Next Steps
- Be sure to join the NLP-CoP if you’d like to stay connected and receive more information about events like this.
Well done for this very informative session and Blog. Keep up the good work in enlightening us along this interesting journey on working with AI.