Ethical AI for Qualitative Data Synthesis: Balancing Promise and Prudence
This post was authored by Grace Lyn Higdon, co-lead of the Ethics and Governance Working Group
As cost pressures drive funders to decrease dedicated resources for a wide range of social research and evaluation, AI is rising to the top of an increasingly limited set of options to address financial constraints. Our recent Ethics & Governance Working Group session captured this central tension facing the social change sector: as AI tools become more accessible, organizations face mounting pressure to adopt them – ready or not.
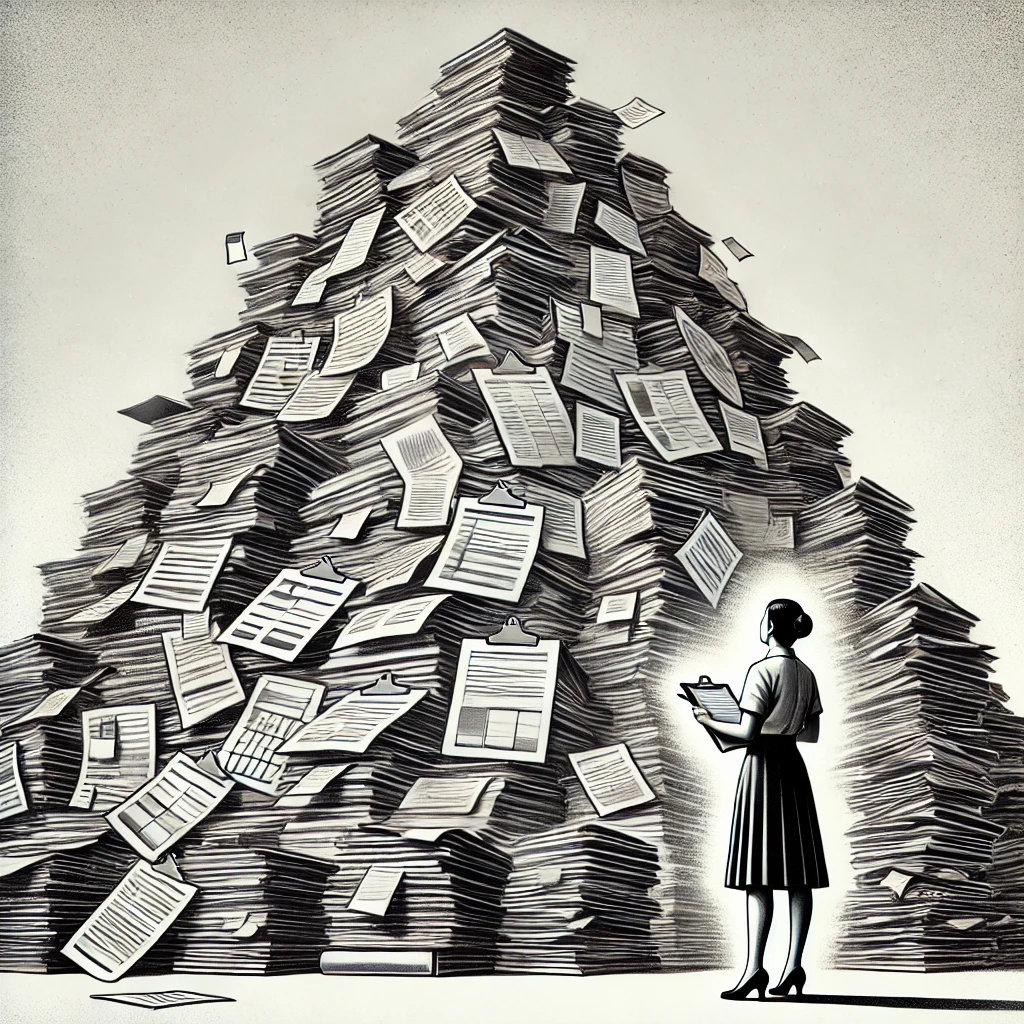
But can AI actually deliver on its promise to help us make sense of mounting qualitative data? And more importantly, should we let it?
While these tools promise to help organizations process growing volumes of qualitative data more efficiently, they also raise important questions about ethics, methodology, and human oversight. The MERL Tech NLP Community of Practice convened a fascinating discussion on the responsible use of AI for qualitative data synthesis on 14 November 2024.
Our speakers brought diverse perspectives from the front lines of AI experimentation: Elizabeth Long, founder of DT Innovation, who has been exploring AI’s potential to surface behavioral change insights; Niamh Barry, Senior Director of Measurement and Impact at Caribou Digital, who tested GenAI for analyzing innovation fund applications; and Steve Powell, co-founder of Causal Map, who develops AI tools for data collection and analysis.
What AI Does Best (And What It Doesn’t)
The panelists found notable agreement around AI’s current strengths and weaknesses. All observed that AI excels at rapidly processing large volumes of qualitative data, particularly when performing well-defined classification tasks against existing frameworks or rubrics. As Steve Powell explained, AI is most effective at “doing a lot of small, focused tasks at scale” where the criteria are clear and most people would agree on the correct classification. Examples of these tasks include applying rubrics and classifying short texts into groups that are predefined.
Additionally, the experts also aligned on AI’s significant limitations. All noted that AI struggles with nuance and context – critical elements for meaningful qualitative analysis. Niamh Barry shared that in analyzing 500 innovation fund applications, while AI could efficiently process and summarize data, “it can disregard at scale important contextual information or miss key narratives.” Elizabeth Long similarly found that while AI could categorize insights into behavioral frameworks effectively, “some of the insights are very vague” therefore the conclusions required significant refinement.
When it comes to making evaluative decisions about larger pieces of text, the picture gets even murkier. As Steve underscored: “If we can’t agree amongst ourselves what it means to answer that question correctly, then the last thing we should be doing is asking an AI to do it[…] We shouldn’t be passing responsibilities onto robots.”
Putting ‘Communities in the Loop’: Human elements are more critical than ever
Rather than seeing AI as a replacement for human-driven tasks, our panelists strongly agreed that human oversight remains essential. AI should enhance rather than replace human processes. For example, Niamh Barry stressed the need for both qualitative research expertise and subject matter knowledge to effectively validate AI outputs.
But this only works if organizations resist the temptation to skip validation and refinement steps. Getting AI to produce trustworthy results requires significant investment in oversight.
It’s rather like having an enthusiastic but inexperienced intern – they might work quickly, but you need to check everything they do.
While the technology can process data rapidly, Niamh noted that their experimentation took longer than traditional human analysis due to the need to validate results and build trust in the system. And as the Ethics and Governance working group has previously discussed, even the scientists who build AI can’t tell you how it works.
Elizabeth Long introduced an exciting new take on ‘human in the loop’*: putting “community in the loop” – suggesting that AI’s rapid initial analysis could free up time for deeper community validation. This would mean returning to a community, showing them the analysis of their conversations, and checking whether the identified themes and insights resonate – “One of the key steps that should in theory always happen in a project, but frequently does not.”
Why does this step often get skipped? Elizabeth pointed to the typical time lag between data collection and analysis. When researchers spend weeks or months processing results, the momentum for community engagement often dissipates or budget constraints might prevent a return visit. By handling initial data processing more quickly, AI could create space for what truly matters: engaging communities while the research is still fresh and relevant. It’s a reminder that efficiency isn’t just about processing speed – it’s about getting to more reliable insights that truly reflect community perspectives.
Key Questions from Participants
The Q&A session discussed the following questions:
- Data Privacy and De-identification
A participant raised crucial questions about handling personally identifiable information (PII) and adherence to GDPR standards. Niamh shared Caribou’s challenges in de-identifying 500 applications, noting that even after manual removal of organizational names, AI tools sometimes still referenced this information in responses. This highlighted the complexity of ensuring true data privacy at scale, a promise made by commercial LLMs that we would be prudent to keep under suspicion as research continues to show how easy it is to trick LLMs into evading guardrails pertaining to PII.
- Cultural Context and Data Types
Questions emerged about AI’s ability to handle culturally specific data and process interview data accurately. Elizabeth offered an interesting perspective, noting that while the analytical process could remain consistent across cultures, the real challenge lay in ensuring the AI had appropriate contextual understanding to interpret the data meaningfully. As Steve described, “It can guess. It can extrapolate a bit. And those guesses and extrapolations might be quite good, but also it will sound convincing when perhaps it’s wrong.”
Clear eyes, responsible hearts
AI tools for qualitative analysis are here to stay, and cost pressures will likely push more organizations to adopt them. But our discussion revealed that successful implementation requires much more than just purchasing a software license.
And buyer, please beware of AI washing leading to your unwitting purchase of AI vaporware. This is a pervasive industry-wide problem when companies overstate or even invent AI capabilities that in actuality haven’t been built or do not exist. There are raging debates about if this current era is a boom or a bubble and if LLMs can in fact reason like some of their evangelists claim. Furthermore, there is consensus forming around the alarming extent of AI climate impacts. All of these issues are extremely pertinent to our sector and the principles, values, and ethics we espouse.
Therefore, social change organizations need to approach AI adoption for qualitative data synthesis with clear eyes. Rather than viewing AI as a replacement for human processes, organizations should focus on identifying specific use cases where AI can enhance existing methodologies while maintaining rigorous oversight and ethical standards. Most importantly, we need to resist the false promise of AI as a silver bullet for resource constraints. As our speakers demonstrated, responsible AI implementation requires significant investment in skills, processes, and validation. The question isn’t whether AI can help us process and synthesize qualitative data – it’s whether we’re willing to make the investments necessary to use it responsibly.
—–
📌 Interested in taking part in similar discussions in the future? Make sure to join the NLP CoP, a community of over 1000 development and humanitarian practitioners working at the intersection of AI, digital development, and MERL.
* “human-in-the-loop” is used in multiple contexts but here refers to the ability of a user to leave an automated, AI powered conversation, and chat to a human, before returning to an automated experience (a front-end function). It can also refer to the role of humans in validating or correcting outcomes created by an AI system (a back-end function).