Exploring the opportunities and risks of ChatGPT: Highlights from the MERL Tech Natural Language Processing (NLP-COP) Meeting
This post was written by Dr. Matthew McConnachie, Evaluation Consultant, NIRAS
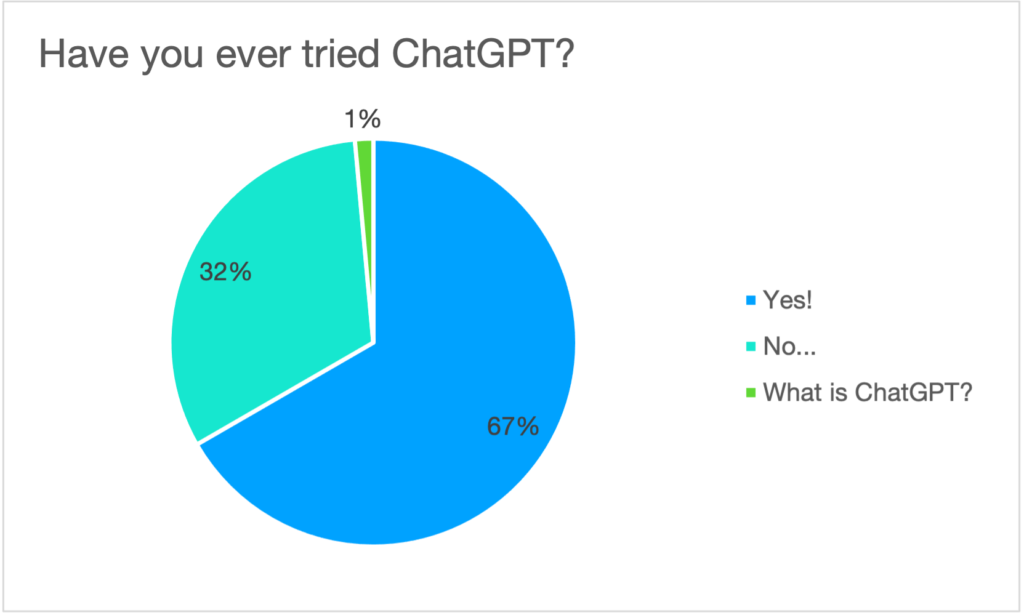
ChatGPT and the AI technology it draws on (large language models) offer huge opportunities to revolutionise the way evaluation is done across the full workflow from data collection to analysis to reporting.
On March 8 we had our second meeting of the Natural Language Processing Community of Practice (NLP-COP) where we explored the opportunities and risks of ChatGPT and similar AI language models.
The meeting included presentations from several people and a lively discussion with the 100+ participants (67% said they had tried out ChatGPT).
Here are some of the key highlights from the meeting.
Overview of the ChatGPT interface and tools
We started with a live demo of ChatGPT, where Paul Jasper showed how outputs are generated based on user prompts in a conversational format (see Paul’s slides here). As shown below, the prompts can include questions or instructions given to ChatGPT. ChatGPT’s outputs can be based either on information it already has access to (from its snapshot of the internet in 2021) or text uploaded to ChatGPT by the user (currently restricted to 500 words, but see below for workarounds).
One of the key strengths of ChatGPT, relative to traditional supervised machine-learning NLP methods, is the power it gives users to develop customised prompts, allowing near limitless options to tailor the instructions given to ChatGPT (e.g. summarising, classifying, generating, analysing and extracting text information). ChatGPT’s capabilities are also continuously improving, recent additions in the release of ChatGPT-4 on 14th March) include the ability to process and respond to uploaded images.
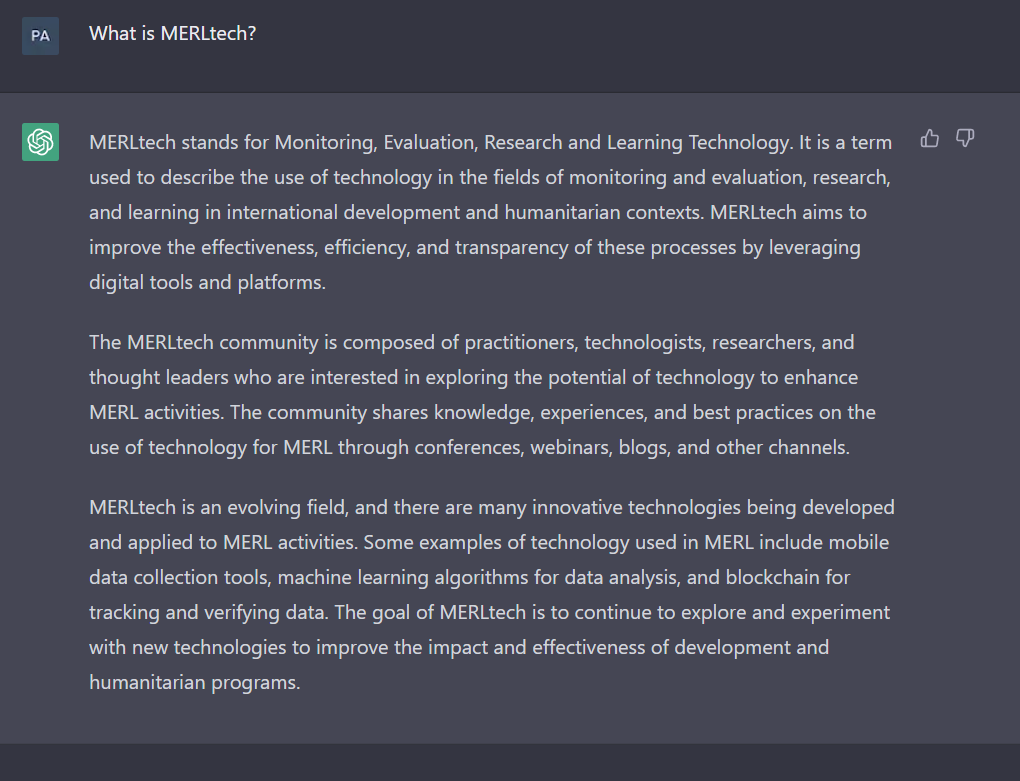
Overview of what ChatGPT can do for MERL practitioners
Rick Davies and Kerry Bruce laid out some of the main areas where ChatGPT and related technology can be used for MERL including:
- Summarising existing text. This can include condensing text or pulling out key points, findings or headlines. Evaluators often have to do this task during the data analysis stage when summarising information from documents or interview transcripts. At the report writing stage, it could also be used for drafting executive summaries and abstracts.
- Comparing multiple texts. ChatGPT can be asked to compare and identify the differences between texts. It can also be asked to rate aspects like which text is more optimistic, likely or realistic. This could be useful for comparing/triangulating different evidence sources.
- Analysing individual texts. This covers a wide range of capabilities from (1) information extraction (actor/place/relationship extraction); (2) text classification similar to qualitative thematic analysis covering both deductive and inductive coding (including sentiment analysis); and (3) causal relationship extraction, the process of identifying and synthesising causal claims.
- Analysing tables and images. ChatGPT can write a supporting narrative to data tables and also images. This could be very useful during both the analysis and reporting evaluation stages. Although ChatGPT itself cannot generate images, free tools already exist for doing this.
- Creating (generating) text. ChatGPT can draft text based on the information it already has in its model (from the internet in 2021). This could be potentially useful for drafting text for an introduction or a context section of an evaluation report. ChatGPT could also be asked to draft text based on text that is shared with it; e.g., drafting a results or discussion section. ChatGPT is very limited with the amount of text that can be uploaded but other options are now being developed (see below).
For more detail about the above see Rick’ slides, blog on ChatGPT and his explorations with the latest version of ChatGPT. And also see Steve Powell’s blog on processing causal claims.
Given the wide-reaching capabilities of the technology to process, analyse, and generate multimodal information (text, tables and images), it’s clear that the technology is likely to have a big impact on MERL in the coming years.
How does the technology behind ChatGPT work?
As the importance of ChatGPT and related NLP technology grows, it will become increasingly important for the MERL community to understand how the technology works so that we can use it effectively and responsibly.
OpenAI’s ChatGPT is built off a special type of AI deep-learning model, called a large language model or transformer, trained on massive amounts of internet text. Transformers were invented by the Google research team in 2017, outperforming all previous NLP models. The technology only took-off the following year when a method called transfer learning was invented. Transfer learning found a solution to the problem of having to train transformers from scratch (at great expense) each time they were used for specific task areas. Transfer learning involves saving the transformers as pre-trained models for re-use on downstream tasks. The fine-tuned transformers generalise very well to new tasks and domains, requiring limited human-based training.
OpenAI’s breed of transformers called GPT (generative pre-trained transformers) excel at generating human-like text but are highly prone to producing factually incorrect text (hallucinations). To address this issue OpenAI developed innovative methods for humans to train and correct the transformers through a method called re-enforcement learning. The model that powers ChatGPT is based on the GPT and re-enforcement learning.
Options for taking the transformer technology to the next level for MERL
While ChatGPT has amazing capabilities, being largely a demo tool, OpenAI’s likely main intention with the application is to raise public awareness and improve the underlying model based on user feedback.
The full transformer-enabled revolution for MERL will come from using the tailored applications that are built on top of the pre-trained transformer models that OpenAI and others have developed (see diagram below). This could include off-the-shelf applications or tools developed for specific MERL projects. The applications will allow for greater functionality e.g. the ability to search and generate text off large document/text archives. For example, Rick Davies found this tool for building custom apps based on OpenAI’s GPT-4, requiring no coding skills.
In the longer term, the MERL community could explore options to build a transformer model from scratch trained on MERL domain relevant documents. This will require collaboration to pool together the text data and cover the cost of training the model (potentially costing millions of dollars). As found in other sectors where it has already been done, the benefits could outweigh the costs.
See my slides on this point here.
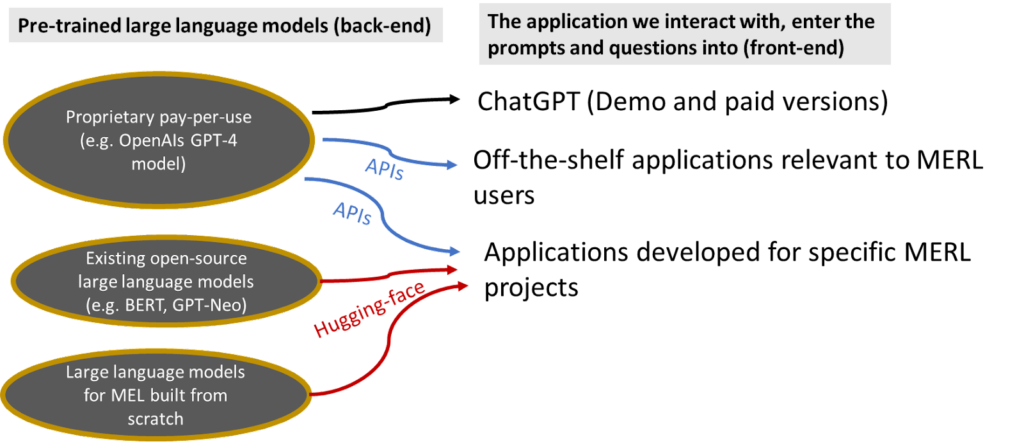
Limitations and risks, ethical challenges
ChatGPT and the underlying technology has important limitations and risks that we cannot ignore. Mark Irura addressed some of these during the meeting.
It is currently impossible to check the facts and logic behind the answers given by the tool. Despite ChatGPT being less prone to hallucinations because of its reinforcement learning, it still generates erroneous content, which is hard to detect. Furthermore, the tool is trained on a snapshot of the internet in 2021, so is not able to factor in current events when generating answers.
Training the tool on the internet and other historical data sets comes with significant risks of perpetuating biases (racism, sexism, ethnocentrism, and more). Although the tool is multilingual, it is trained on the largely English speaking Internet and English-language texts, with English speakers’ cultural biases attached.
The first two issues are likely to be solved through the development of bespoke applications (as we note above), the other issues are more fundamental and will require coordinated effort from the MERL community to address.
Models like ChatGPT won’t be replacing evaluators any time soon, but the technology will definitely be used for helping us do our work. We need to be aware of the limitations and risks, especially given the work that our sector does and our attention to inclusion, fairness, participation, and other values that tools like ChatGPT do not fully enable.
Next steps for the NLP Community of Practice
To close out the meeting, Megan Colnar and Linda Raftree shared what’s next for the NLP-COP.
There has been a huge amount of interest – far beyond what was imagined when the call for our January 19th kick off meeting went out. To date we have about 250 people who have expressed interest in the COP and many others who want to participate at a less involved level, for example attending periodic webinars.
A small group is working behind the scenes to create structure for the NLP-COP. We have developed a Charter that lays out options for different levels of engagement (non-members, members and those who want to start or join specific working groups) and provides an overview of the NLP-COP-s goals, values, and ways of working. We will ask people to sign onto the Charter in order to join as a NLP-COP member. Key points include a focus on ‘real world’ problems that those working in the ‘global South’ want to tackle, diversity and inclusion, and a commitment that anything the COP or its working groups produce will be open and public goods.
We’ll be sharing the Charter with everyone on the current list of NLP-COP members and asking people to sign on. Sign on as a member will come with greater access to NLP-COP assets and opportunities to join additional meetings and convenings, access to the Linked-In discussion group and the NLP-COP Slack, a ‘help desk’ for NLP and MERL queries and support, and other benefits.
We’re participating in a symposium hosted by New Directions in Evaluation (NDE) on March 24, and have submitted an article to this journal on the ethics of NLP in evaluation. We’ll also be at the American Evaluation Association (AEA) and UK Evaluation Society (UKES) conferences later this year and plan to host an in-person gathering. In 2024, we’re planning for another MERL Tech Conference, which will focus on AI and Machine Learning for MERL.
So far this work been at a volunteer level, and the NLP-COP would benefit from funding so that we could provide the support that COP members are asking for in a more quality and timely way. If your organisation is interested in funding the NLP-COP, please do get in touch with Linda – we have a concept note ready to share!
If you’d like to join the NLP-COP, send an email to Linda with the subject line “Join NLP-COP”
Leave a Reply
You might also like
-
Meet us (virtually!) at RightsCon next week
-
Getting Real About Artificial Intelligence: GenAI, Evaluation in International Development, and the Case for Caution
-
Key takeaways from our first Gender, AI and MERL Working Group meeting
-
What are the potential benefits, considerations, and risks of AI for Research Funding Organisations (RFOs)?
Fascinating information sharing on developments of AI and Evaluation. I would love to hear more…. Pease include me with updates, conferences, webinars etc.
Très instructif et très important pour le métier d’Evaluateur