Getting Real About Artificial Intelligence: GenAI, Evaluation in International Development, and the Case for Caution
Written by Matt Klick via LINC.
Each time I sit down to write about what I’m seeing in Artificial Intelligence (AI), development, and evaluation, I feel like I’m looking outside a high-speed train window—the landscape whizzing by in a blur while I try to pick out individual mailboxes, trees, or a street sign. New books, new blogs, and better technologies emerge overnight, and clients and colleagues race to embrace and tout the power of yet shinier gizmos while the promise of the technology itself seems taken as inevitable.
I certainly get the hype. I am taken aback by the technology, its availability, and its potential, and Chat GPT, Co-Pilot, and Claude, meanwhile, have become familiar tools of my own to query for background research, summarization, or troubleshooting. So I’m no technophobe, but as a “development professional,” evaluator, and political economist at heart, there has been an internal wince at the breathless enthusiasm I see over AI and evaluation and development, even as I embrace its immensity and appeal.
Why the Long Face?
I’m mainly talking about Generative AI, and the bots referenced above. This would also include image and video generating bots which the user prompts for a desired outcome. Discriminative AI has been around for decades and remains a hugely powerful tool for research and analysis. While the data sets used in activity evaluation are generally too small to leverage AI and machine learning to determine complex relationships and patterns, they are exceptional at dealing with big, unstructured data and could be—if targeted appropriately—groundbreaking in addressing global development conundrums, including issues as complex as democratic backsliding, political unrest, malnutrition, public health crises, and climate resilience.
Generative AI—revolutionized by the advent of transformers and large language models (LLMs)—requires vats of computing power (and thus real-world energy during a climate crisis, it’s worth noting) but currently excels at quickly resolving simple tasks and crunching overall limited amounts of data. The ability of the current models to mimic human intelligence, and their accessibility, make them incredibly appealing to those of us confronting a pile of interview transcripts and a tight deadline.
On Evaluation Integrity
My misgivings in some ways started with such a task. But with industry best-practice being replicability, and at least transparency in demonstrating how findings were derived and validated, the black box conundrum of using generative AI to analyze transcripts, I quickly realized, was unavoidable. While a “human-in-the-loop” (HITL) approach has emerged as a new “best practice” of some kind, it is unclear to me whether this is a realistic safeguard. A human may flag purely erroneous outputs but it can be difficult to gauge the accuracy of plausible outputs, and thus the time savings when all outputs require validation are unclear, especially when dealing with nuanced qualitative data.
Other concerns in this regard are the inherent biases, well-documented at this point, in the LLMs that are being leveraged for analysis. In effect your bot of choice will bring a “world view” – the warped one of the internet and its training data no less – to its analysis of the data.
Personally Identifiable Information (PII) is another massive concern. Without a safeguard on your end to ensure that your bot is not using the data for ongoing training, you risk exposing PII, when you likely assured someone you would not. Even if de-identified, data aggregators can triangulate details well enough to determine identities—a considerable risk anywhere, but especially in anti-democratic environments with increasingly sophisticated surveillance capacities. (Other observations and concerns of AI in evaluation, from a recent MERL Tech Community of Practice webinar, are detailed in this article.)
On Localization
AI is currently anti-localization. A small, poorer country’s Civil Society Organizations, scholars, farmers, and activists can indeed access and utilize generative AI for their own ends, like anywhere else, but fundamentally this is a blunt tool, subject to error, for time savings, and hardly fosters a deeper renegotiation of power dynamics.
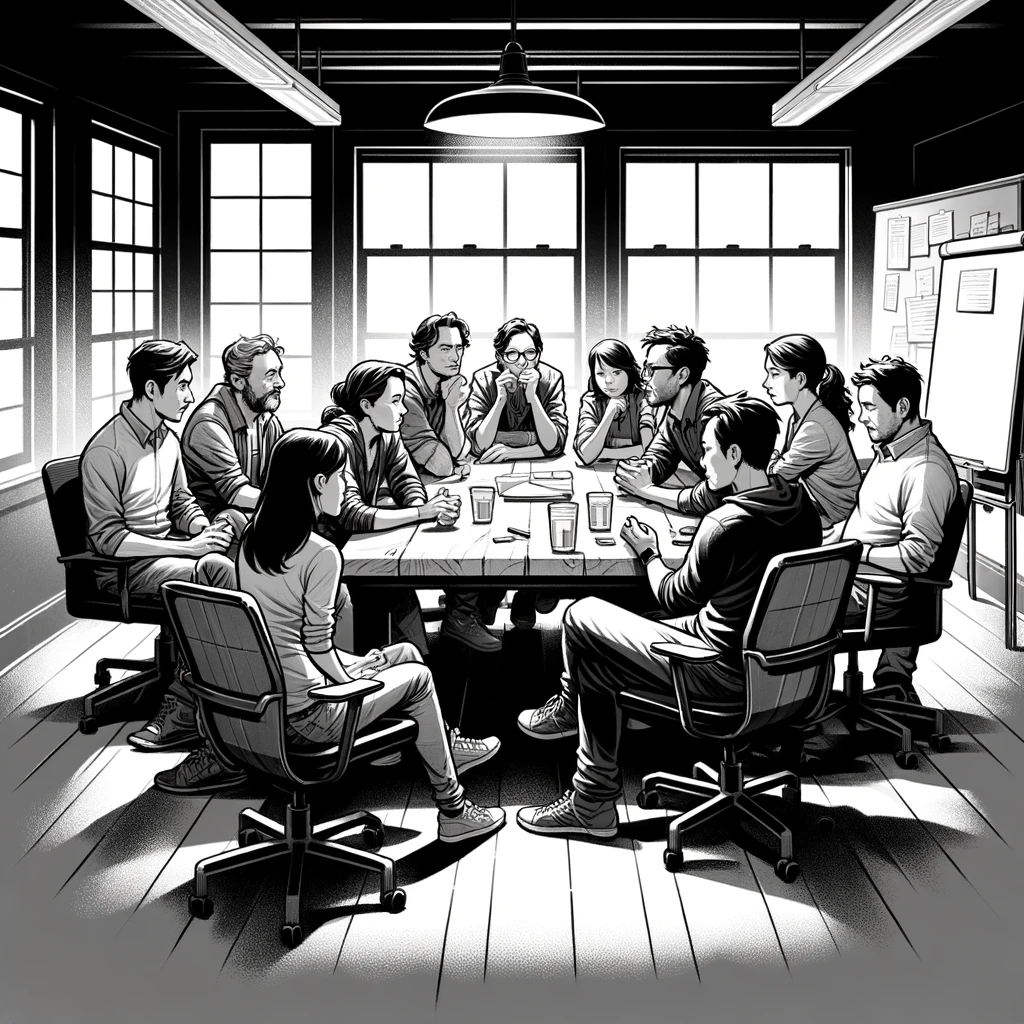
If localization means leadership, agency, and at least consultation in the development of a tool that is shaping economies, democracies, and livelihoods worldwide, then the diffusion of generative AI is more harmful to localization than not. As already noted, it is trained on Western-centric data, reflecting the power dynamics and sexism therein, as well as the stereotypes, inaccuracies, and misinformation that finds a disproportionate sized home online. As an example of the disconnect, minority languages in particular are susceptible to being misrepresented, and represented poorly.
While local ownership of AI is not impossible, the data, models, hardware, and even raw power for sprawling data centers are currently housed principally in the United States, even more narrowly Silicon Valley. And for the time being, more of us are subject to AI rather than its master, through super-charged disinformation that shapes electoral outcomes, spreads violence, and through government surveillance.
The Ethical Divide
Artificial intelligence is a function of real intelligence. More precisely it’s a function of a poorly paid, sometimes exploited workforce that labels data for machine training. The annotation industry, perhaps indicatively, is not housed in Silicon Valley but is fundamental to its success. And given our safeguarding and Do No Harm commitments, I’m unsure that generative AI even passes muster.
Anecdotally, when I asked a titan of the evaluation space their feelings about this, I was somewhat crestfallen when they brushed it aside as some sort of inevitable externality and a temporary necessity for the magic that it yields (in this particular instance the “magic” being a mostly cartoonish image that was generated by in some ways pirating a real artist’s work). This instance underscores just how powerful the allure of generative AI is to even the most seasoned researchers and evaluators, whose skepticism I had thought was instinctive. It is this repeated enthusiasm, in fact, in class after class on prompting, or AI for evaluation, that is what has me most concerned.
Perhaps not most concerned. That would instead be the data privacy of the most vulnerable in an era when it is this very personal data that has emerged as a sort of electronic lithium—a raw ore which enrichens the handful of elite organizations that currently dominate AI. It would be the “AI capitalism” emerging in which a hyper elite set of organizations extract rent from their domination of AI globally. It is our again misplaced faith in their “voluntary ethical behavior” to do what is right, and just, and equitable, when ultimately the incentive is monetization, even if that means violating previous ethical commitments (and when the recent internal efforts to police AI resulted in wreckage).[1]
An Appropriate Skepticism
While entities within development are grappling with what safe and ethical use of generative AI might look like or require (see also here, in fairness), the colonization of Generative AI, risks to data privacy, and risks to evaluation work stemming from error, let alone methodological ambiguity, are not going away soon, and I do not see a sufficient wrestling with these issues by our industry.
There are things the rest of us outside Silicon Valley can be doing, whether to guard ourselves and our use with professional standards and regulations, including those that reference our existing obligations to safety. Another, more difficult and longer-term effort will be to welcome and encourage the development of models and ownership of computing power in the Global South, and more granularly there are democratization approaches we can potentially adopt. In the meantime, a minimal place to start is with a healthy dose of renewed skepticism about generative AI for evaluation, even if we adopt it day-to-day for the appropriate task. The time for breathless enthusiasm, at any rate, has come and gone.
[1] See also here: “I started crying”: Inside Timnit Gebru’s last days at Google | MIT Technology Review
You might also like
-
Welcoming our new AI+Africa Lead for the NLP-CoP: Vari Matimba
-
Do you see what I see? Insights from an inclusive approach to AI ethics governance.
-
New guide: key questions to ask before using GenAI for research on violence against women
-
RightsCon Recap – Assessing humanitarian AI: what M&E frameworks do humanitarians need in the face of emerging AI?