How can we make the most of GenAI for SBC?
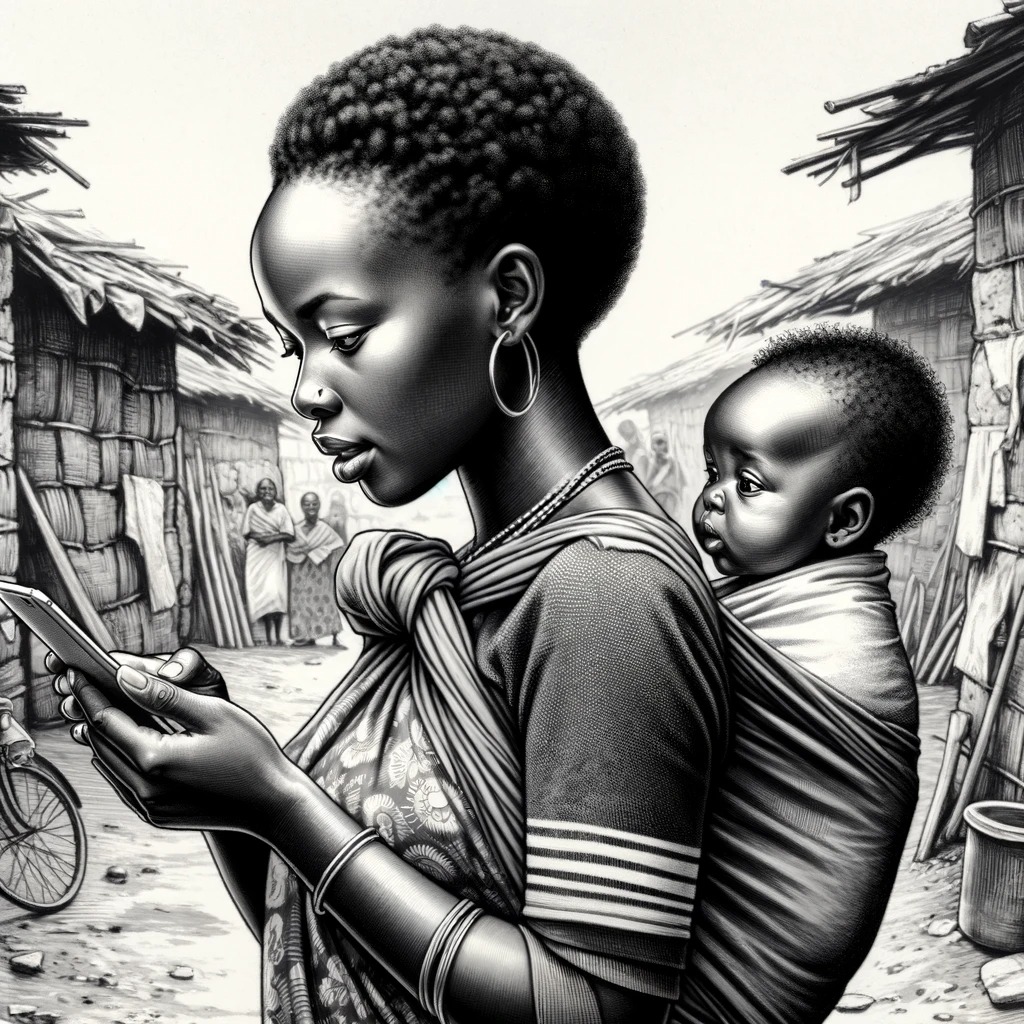
This post was also published on the iMedia Associates website.
Predictive algorithms, Natural Language Processing (NLP), and conversational interfaces such as chatbots have been playing an increasingly important role in the deployment of digitally-enabled Social and Behaviour Change (SBC) programmes for the last decade. The release of ChatGPT by OpenAI in November 2022 heralded the beginning of a new era in digital programming.
Unlike predictive AI, generative AI (GenAI) uses Deep Learning (an advanced form of Machine Learning) to identify patterns in extremely large data sets in order to generate entirely new data in the form of text, images, audio or video. The content generated comes close to mimicking that created by humans, opening up many new possibilities for SBC programming, as well as producing new challenges and reinforcing existing ones.
As was the case when other digital technologies and tools such as SMS/text messaging, Interactive Voice Response (IVR), mobile web, apps, social media and chatbots first emerged, SBC practitioners and researchers are faced with many questions before they can decide whether to leverage GenAI as part of their programming – let alone how.
To this end, The MERL Tech Initiative, on behalf of iMedia Associates, took advantage of the 2024 ICT4D conference held in Accra, Ghana in March to convene some 50 SBC and digital development practitioners from over 30 organizations for a 1-day workshop where we began collaboratively developing a research agenda related to SBC and GenAI.
How could GenAI be useful in SBC?
The GenAI moment is unique because there is little to no time lag between our own discovery and exploration of the technology and our efforts to deploy it as part of SBC programming. In comparison, many practitioners had already been using mobile web, or instant messaging, long before it became feasible to use these as channels for reaching community members in the global south.
This means that we are wrestling with our own personal and internal uses of the technology while we figure out how to use it within programming. In the rush to use GenAI, we might fail to pause and reflect on the many ways a new technology can be used, focusing on only the most hyped use-cases.
During our consultation, we therefore took some time to discuss how GenAI could be used across the whole spectrum of programme activities, and how practitioners were already using it, or planning to use it.
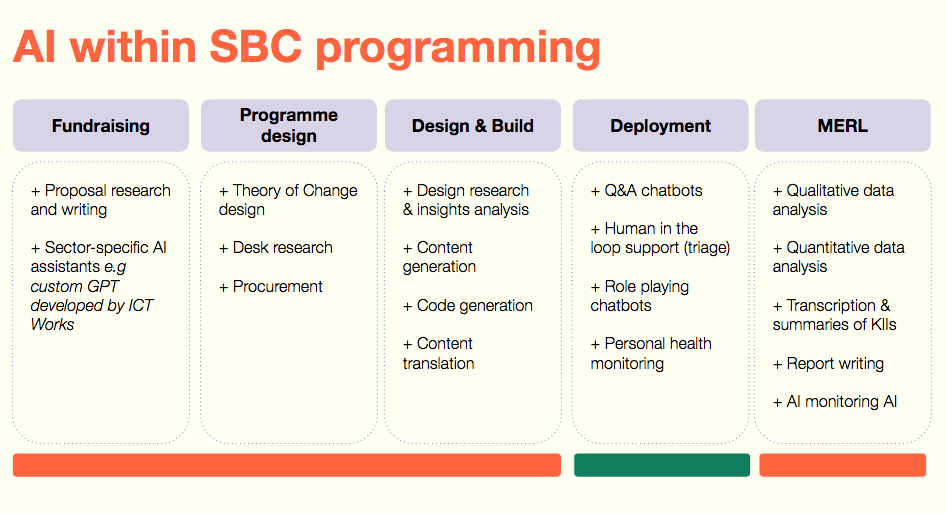
Examples of use (sometimes actual, but mostly potential) covered
- programme development & operations (proposal development, managing documentation to support decision-making, research support & gap identification, internal working groups and governance, content generation)
- community-facing products (Conversational assistants / interfaces, for example a voter education chatbot to support free elections and combat disinformation; triage support before signposting to human led services (for example for Sexual and Reproductive Health services), misinformation/fake news tracking, education support for school children e.g Rori by Rising Academies);
- language-based applications (creating models in native language for example by Data Science Nigeria); using GenAI for agile translation); and
- MERL (Social media and feedback data analysis including sentiment analysis; general data analysis; performance analysis of products or interventions; voice data analysis of call center interactions (for example, Family Planning support conversations); automated services for post-intervention follow-up; managing feedback and accountability to program participants; developing M&E frameworks and designing M&E plans.)
Interestingly, many participants did not have a strong understanding of Generative AI beyond the existence of ChatGPT and other conversational assistants, signposting the potential for missed opportunities and risks.
Don’t panic! The GenAI moment in SBC hasn’t really started
Although the workshop was convened for the purpose of developing a research agenda, what emerged quickly was that most practitioners and researchers were not ready to articulate complex research questions. Rather, they were seeking guidance, training and capacity building on if, when, and how to effectively and responsibly implement GenAI.
They were also interested in accessing case studies of existing applications as well as data that could be used to design and benchmark their own indicators of adoption, engagement and impact. Interest in organizational guidance (on when/when not to use Gen AI) and “how would we get started” figured heavily in our discussions. Tellingly, very few examples of live products supported or powered by GenAI were flagged.
We did identify a range of research and evidence needs and big questions, particularly around data rights and privacy, and the risks posed by the bias and potentially unreliable content generated by LLMs. It’s worth noting that many questions were similar to those practitioners have asked at the peak of earlier ICT hype cycles.
We need guidance and more capacity
Guidance and capacity building requirements raised by participants included the need for resources on:
- Getting started with GenAI within SBC programming: What could I be using it for? What’s the first step?
- Using GenAI within programmes: how do I implement it? What skills do I need? What external organizations might I need to work with? How long will it take to be up and running? How much is it going to cost?
- Data, privacy and wider safeguarding aspects: What do I need to know? What do I need to plan for? How will my existing policies and processes need to change? What could go wrong and how do I mitigate the possible risks? What data can I put into different kinds of chatbots?
- Ethics: How can I implement a GenAI intervention ethically? What ethical considerations do I need to know about? What guidance exists already? Who is accountable for GenAI interventions?
- Localisation, relevance, trust: How do I localize and contextualize my GenAI intervention to mitigate bias and improve relevance for my end users? How much does this influence uptake and impact? How do I build trust and confidence in my GenAI service?
- M&E: How do I evaluate a GenAI powered intervention? How can I use GenAI within evaluation activities?
- In-house uses of GenAI: How can I support staff in their own use of GenAI in their work? How do I need to regulate this? What policies should be in place internally? What about for partners, grantees, subcontractors, and vendors? How do I know if GenAI has been used for something?
We also need to begin building up good practice and evidence
While we attempted to develop more granular research questions, what emerged was the need for illustrative case studies that could help difficult to grasp concepts come to life. In particular, practitioners are seeking clear examples of “good’ vs “bad” implementations, especially stories of early failure. Practitioners concerned with gender inclusivity were especially keen to understand what examples of gender-inclusive or feminist tools already exist, and/or what guidance and tools to create to evaluate GenAI through this lens.
Similarly, cost-benefit analyses comparing time and budgetary efficiencies in GenAI vs non GenAI interventions were seen as crucial. One of our speakers, Maria Dyhsel from Tangible AI, shared that whilst GenAI responses within their Syndee chatbot pilot were more flexible and responsive than those provided by their predictive model, leading to longer conversations, the engagement was not necessarily more productive (for example based on exercise completion); and whilst time was saved on conversation design/scripting, more time needed to be spent on guardrails, moderation and red-teaming. Small insights such as these were greeted with much enthusiasm, underlining (again) the need for a culture of data transparency.
Indeed, practitioners raised the urgent need for cross-sectoral benchmarking studies and the fostering (by funders especially!) of a transparent data and knowledge sharing environment to support the development of realistic indicators of reach, adoption, engagement and impact. At the level of risk-assessment, we also discussed the need for case studies examining the financial, environmental and ethical costs of using GenAI, to help support funders and grantees alike to decide if it is advisable to proceed.
Beyond these requirements for case studies, some interesting early-stage research questions did emerge. These were broadly focused on two workstreams, namely programme/service/content design, and MERL activities.
Questions that feel particularly pressing were concerned with community readiness and digital literacy for GenAI powered / supported services (How do users understand and conceptualise GenAI? Do they trust it? Can there be such a thing as informed consent relating to data privacy in the age of GenAI?), with an emphasis on understanding how this may differ along generational, gender, socio-economic and geographic lines.
Other research areas relating to impact evaluation would gather evidence as to whether the increased personalisation, and therefore relevance, of GenAI powered services such as chatbots, would actually increase adoption and impact compared to say, a decision-tree style chatbot. Similarly, MERL practitioners working in SBC programming would like to see hard evidence on the extent to which GenAI really speeds up the emergence of reliable insights from programme data – an important cost and efficiency question which would have significant ramifications for day to day practices.
How you can get involved
Whether you work at a funding or grant management level, as a researcher, or at the coalface of SBC programming, you may be wondering what your role could be in addressing some of the needs expressed by the digital SBC sector. Luckily for you, we’ve identified at least 6 specific actions which you could start supporting, right now!
- Help us map existing community-facing and internal uses of GenAI, for example by submitting them to the NLP CoP directory or by sharing them on our Slack channel, to help colleagues understand what “GenAI in SBC” actually means.
- Develop or support the development of varied, easy to understand case studies which dig deeper into some of the questions raised above.
- If you are already working with GenAI, join together with other practitioners to discuss and disseminate your insights, however small, for example at one of the NLP CoP working groups or by volunteering as a speaker.
- Join the SBC working group to help us to develop capacity building resources to address the “GenAI and SBC 101” type questions raised here.
- Hustle for an end to data and insights silos: be bold and put your M&E data (especially your failures) out there in the public domain. Funders, consider innovative ways you could encourage grantees to do this rather than feel in competition with one another.
- If you are considering using GenAI in a community-facing fashion, consider first implementing digital literacy research and training with end users to make sure they are as well-equipped as possible to make informed decisions when it comes to their use of GenAI powered tools and the ethical implications that could entail.
Join us on May 21 for the next NLP-CoP’s SBC Working Group meeting
If you have your own thoughts to add on this topic, you can join the NLP CoP SBC Working Group’s next meeting where we’ll be hearing from 2 organizations already using GenAI in their work.
***
📌 Join over 600 development and humanitarian practitioners in the NLP CoP convened by The MERL Tech Initiative
📌 We need funding to enable the SBC Working Group and its members to respond to the various questions raised (both practical and theoretical). Learn more about how you can support the NLP-CoP and its working groups. If you are interested in sponsoring or supporting this work, please get in touch – we’d love to discuss this!
You might also like
-
Key takeaways from our first Gender, AI and MERL Working Group meeting
-
What are the potential benefits, considerations, and risks of AI for Research Funding Organisations (RFOs)?
-
We Need More Design Thinking in Monitoring, Evaluation, Research & Learning. Here’s how.
-
We’re hiring: Join MTI as our new AI+Africa Lead for the NLP-CoP