Is it even possible to procure an ethical AI tool? Insights from the NLP Community of Practice
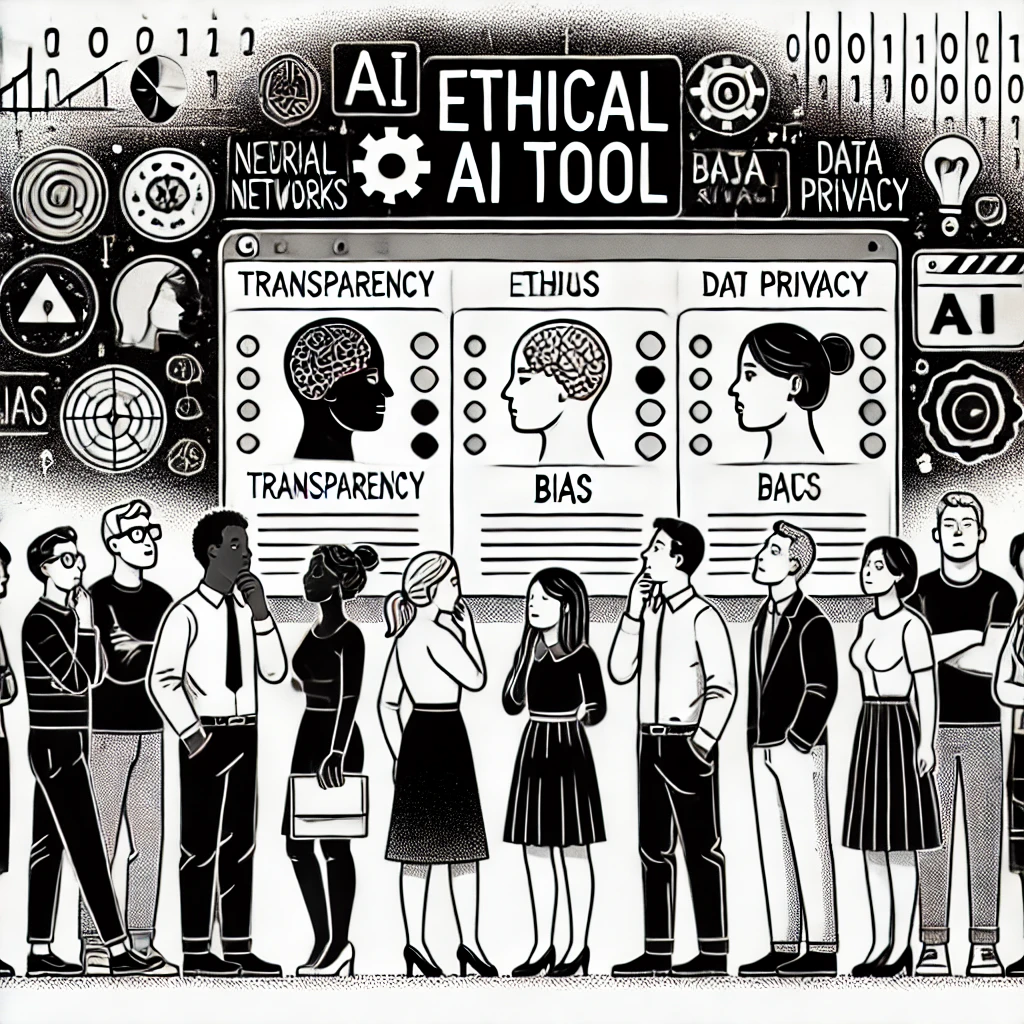
On Thursday 18th July the Ethics & Governance Working Group convened our first live chat on Slack – an informal way to revisit conversations started during our webinar-style events.
3 questions raised by our members felt especially worthy of more discussion, as they cover 3 significant aspects of the use of AI within development and humanitarian work – namely:
- What’s it actually like to try and identify an ethical AI tool as part of procurement?
- In terms of ethics & safety, what should I take into account when doing formative/landscape research using AI powered tools?
- Community members’ perceptions & understanding of GenAI tools – who has feedback and insights from global majority users?
Participants ranged from Behavioral Designers experimenting with using AI to generate insights from qualitative data, children’s rights experts concerned with the use of AI targeting children, data scientists developing AI tools, and MEL experts. If you want to be part of the conversation next time, join the NLP CoP and sign up to the Ethics & Governance working group here (and if you’re on our Slack channel already, you can still access the chat!)
In this first recap, I’ve summarized the insights that emerged on the process of procuring an ‘ethical’ AI tool. This is probably one of the most pressing issues facing organizations who have decided they want to leverage Gen AI for either internal purposes (knowledge management, MERL activities…) or external ones (educational chatbot, survey tool).
Procuring ethical AI tools: a gloomy outlook
One of the biggest challenges our sector faces is that, whilst more and more guidance is being published on the types of qualities an ‘ethical’ vendor or platform should possess or promote, including transparency, explainability, and explicit efforts to combat hallucinations and reduce bias, actually putting this into practice is nigh-on impossible. I can personally testify to this – in response to ethics guidance I produced with the MERL Tech Initiative for an INGO, the client wrote (I paraphrase) “it’s great to know that this is the gold standard… but I still don’t see how I can implement this practically”.
Given that even the makers of LLMs openly acknowledge they don’t always understand how neural networks have used the data they’re trained on, let alone how to express that in terms that can truly be understood by their end-users, many participants shared the sentiment that choosing a truly ‘ethical’ tool, was currently impossible. The ambitions of ethical AI have not yet been matched by reality.
This is made worse by the proliferation of ‘vapourware’- products which are being promoted but that, similarly to Elizabeth Holmes’ Theranos, don’t actually exist yet, or worse, deliver unreliable and unsafe outputs. There was also the suspicion that many organizations have been quietly giving up on anything but the most cursory due diligence, and were as a result not sharing potentially useful insights for fear of being accused of a lack of integrity or thoroughness. A big factor in procurement was legal rather than ethical, for example in the case of one participants’ choice of Azure vs the OpenAI API as it allowed them to be GDPR compliant.
5 steps to selecting the least-worst AI tools
Nonetheless, participants who had gone through the procurement process did share a number of useful tips which, whilst not ‘gold standard’ in terms of responsible AI, could inch us closer.
- Is your organization ready? The first has nothing to do with AI and everything to do with general digital best practice: participants stressed the importance of starting with an honest assessment of organizational readiness for implementing and supporting the responsible use of AI. Does enough staff have the AI literacy to support its use? Are there enough funds in the pipeline to enable through monitoring and safeguarding practices? If not, focus first on building this literacy and avoid building castles on sand.
- Do you really need AI? Similarly, make sure that you have conducted a proper needs assessment, asking honestly IF you should even use AI, let alone where and how, with a risk assessment to back this up.
- Is it Vapourware? Next, be suspicious of overly optimistic pitches – prioritize vendors who acknowledge the limitations of what we can know about LLMs’ use of data and accuracy, and who emphasize the importance of having a ‘human in the loop’*, and who encourage you to start iteratively. A good exercise is to request the T&Cs and privacy policies of the vendors, and assess their willingness to talk you through it in a way that doesn’t feel like they’re obfuscating. You could probe for example on what measures they have taken to protect their most vulnerable potential users – namely children.
- Is Open Source an option? Another alternative which we discussed was prioritizing open source AI promoted by the likes of Mozilla and Mistral. But we acknowledged that users themselves can be intolerant of the shortcomings of open-source platforms which can often deliver a sub-par experience to the commercial models they’re increasingly using, even though they may gain traction in the long run. We wondered whether any attempt had been made to compare the ‘ethicality’ of the big LLMs, including Open Source ones, in a similar way to how the FairWork project has ranked digital platforms for labor and value chain fairness, assessing not just the gains in terms of ethics, but the losses in terms of performance.
- Are Small Language Models an option? Finally, one of our participants pointed out that in our search for more ethical alternatives, we shouldn’t forget the role of Small Language Models (a term I hadn’t actually encountered before!). They do the same as an LLM (understand inputs and generate new data) but are less complex. They rely on less extensive data sets, take less time and resources to set up (therefore less environmental resources), are more easily adaptable, cost less, have lower latency, and are more controllable by those who set them up.
This discussion was not an exhaustive review of the evidence, yet drew on the expertise of a wide range of practitioners actively engaging in this process. The consensus seemed to be that currently, no LLM exists which ticks every box of what ethical, responsible AI should look like. The best we can do is identify ‘the least bad’, using criteria which to a large extent will be specific to the particular mandate of any given organization, rather than following a universal set of rules of what ‘ethical’ looks like. As one participant pointed out, identifying an ethical AI tool is an ongoing process that doesn’t end with the selection of a successful vendor. It’s “an ongoing process that requires continuous evaluation and improvement.” If your organization can’t commit to that, then don’t move forward with AI.
* 📌 Interested in taking part in similar discussions in the future? Make sure to join the NLP CoP, a 700+ strong community of development and humanitarian practitioners working at the intersection of AI, digital development, and MERL.
* “human-in-the-loop” is used in multiple contexts but here refers to the ability of a user to leave an automated, AI powered conversation, and chat to a human, before returning to an automated experience (a front-end function). It can also refer to the role of humans in validating or correcting outcomes created by an AI system (a back-end function).