Key insights on the role of Natural Language Processing (NLP) in helping combat corruption
Written by David Sada (Accountability Lab Mexico) & Bao Han Tran Le (Accountable Now). This is a cross post that was first published on December 7 on Accountable Now’s website.
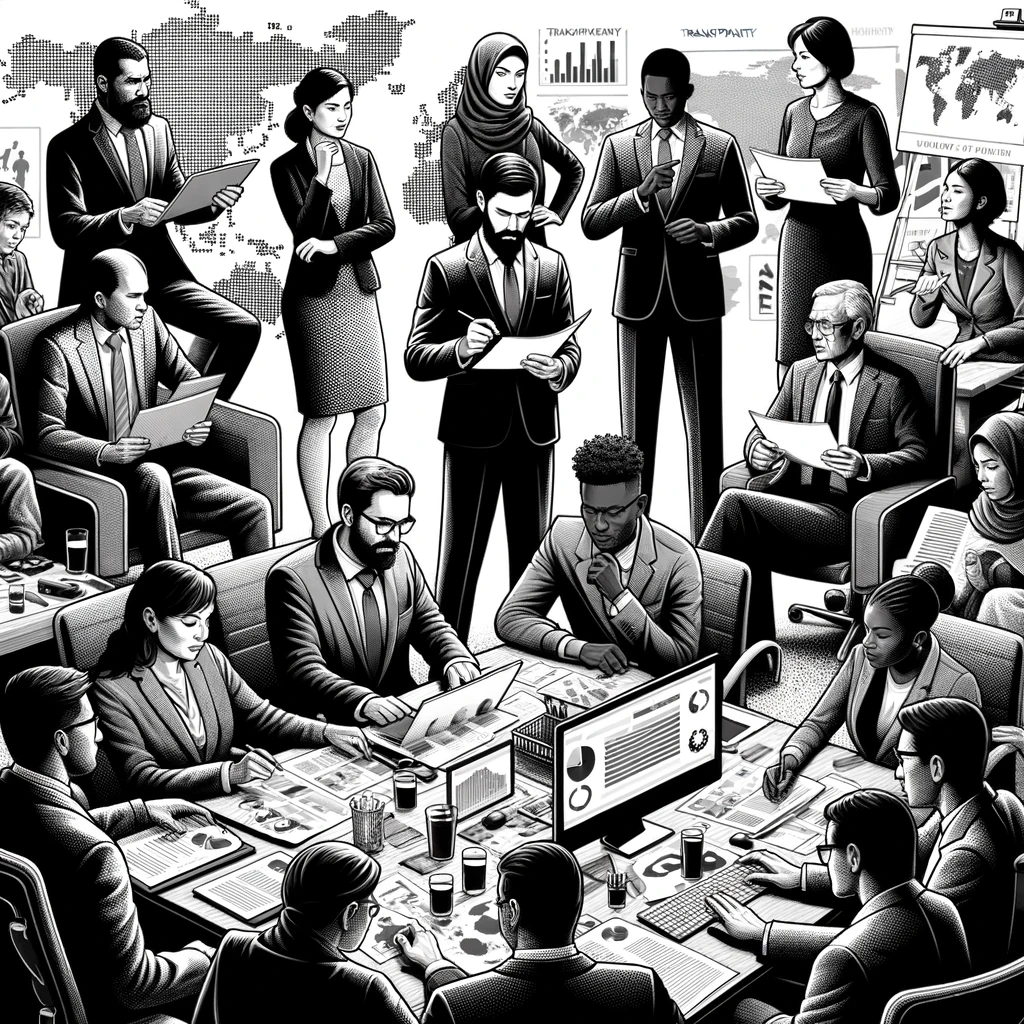
Accountable Now and MERL Tech recently hosted an event with Accountability Lab as part of the Participation and Accountability Working Group (P&A WG) of the Natural Language Processing Community of Practice (NLP-CoP). The event is part of a speaker series, and features insights on the #HackCorruption program from Cheri-Leigh Erasmus, Co-CEO and Chief Learning and Agility Officer at Accountability Lab and David Sada, Operations Manager at Accountability Lab Mexico.
#HackCorruption, developed by Accountability Lab in collaboration with Development Gateway and the Centre for International Private Enterprise (CIPE), brings together professionals from the fields of technology, data science, civil society and policy-making to develop innovative solutions to combat systemic challenges of corruption; in doing so, they create, refine and build upon existing systems that seek to improve transparency and accountability while strengthening integrity within governments.
From their reflections, what was apparent is that Artificial Intelligence (AI) and Natural Language Processing (NLP) have the potential to be an important tool in the development of these systems, which needs to be further explored. The diversity of contexts, implementations, and creative solutions that have come about throughout #HackCorruption provided unique perspectives and key learnings on NLP-related themes; these include institutional readiness, data limitation and under-representation, and civic engagement.
Institutional Readiness
Despite NLP tools’ potential to improve a broad spectrum of institutional processes, success remains dependent on interested institutions’ context and capacity. As NLP is a novel and exciting tool, using it without clear resourcing or capacity may lead to failure and future resistance towards innovations. Key considerations are therefore needed:
- Defining clear objectives for development will help define a clear workflow and assess needs and capabilities of the teams involved.
- Be ready to iterate. Deployment of these projects require time, resources and effort to build and improve.
- Continuity is key; NLP is dependent on continuously feeding into the information systems of which it is built upon.
- An informed and diverse consulting team both internally and externally is essential to provide wide ranging perspectives on how solutions may affect different groups and localities. But also is a key resource to identify and resolve potential gaps in data as well as biases inherent to the system.
Global consensus on reference points and standards for institutional adoption remains in progress, but the variety of emerging projects aimed at tackling them are encouraging. As we continue to advance, clear guidelines, systems and reference materials must be collectively built as a public good to ensure responsible uptake of these technologies.
Data Limitation and Under-representation
NLP is only as good as the data that it operates on. Therefore it is important to view NLP as part of a wider process that involves assessing the foundational dataset, ensuring that its collection, structuring and standardization are carried out with accuracy. Institutions must ensure that the data they are using is representative and respects the rights of the population whose data is being collected.
Representation is furthermore important as under-representation can create limitations, widen the digital literacy gap, and expose vulnerable populations to an additional layer of structural violence (e.g discrimination through social security systems, automated hiring protocols, or healthcare assessment) that can aggravate inequality and limit institutional accountability. It is therefore imperative to guarantee the safe representation and inclusion of marginalized populations throughout the data cycle.
A crucial first step is to establish data policies that are clear, concise, accountable, and transparent. This ensures that the advancement of these technologies does not contribute to exclusion, extraction, and corruption.
Civic Engagement
It is increasingly important that we collectively build clear guidelines, systems and reference materials as a public good for responsible uptake of these technologies. This serves as a huge opportunity for organizations across all fields to participate in educating, improving governance in projects that involve the use of NLP.
Open data is in essence a public good, and should be treated as such. Civic engagement can provide a unique opportunity for collaboration in improving data infrastructure, and can offer localized perspectives on how to deploy, refine, and implement best practices for NLP systems.
In essence, NLP tools represent a powerful opportunity for innovation in civic engagement. Each new project, as we have seen in the Hack Corruption program, brings unique and broad perspectives that can help drive forward how we safely integrate and develop new practical approaches for usage of powerful language models and as we have learned, deployment of NLP solutions in itself should never be a goal for any organization, it is an extremely powerful tool that should be treated as such.
We hope more organizations see the potential that even basic use of NLP can bring to certain processes. They are not perfect by any means, but it is essential that we bring as many visions as we can to guarantee representation and discover the platitude of ways in which diversity can only help them improve and together overcome the challenges we face.
The #HackCorruption program and insights shared by Accountability Lab underscore the transformative potential of NLP in combating corruption and promoting accountability. However, its responsible use requires continuous collaboration, clear guidelines and a commitment to addressing ethical considerations. Additionally, NLP remains as one tool within a diverse kit; it is not a miracle cure, and other tried and tested methods and tools should not be swept aside in favour of a novel ‘solution’.
Interested to learn more? Check out:
- Accountable Now’s dynamic accountability approach which supports organizations to apply a participatory and inclusive lens throughout their work.
- The NLP-CoP (hosted by MERL Tech) to explore the use of NLPs for Monitoring, Evaluation, Research and Learning purposes.
- If you want to know more about our work at Hack Corruption.
- Some examples of the diversity of projects we receive.
- If you want to learn more about open data and its reaches.
- If you are interested in learning more about representation and decolonization in data.
You might also like
-
Welcoming our new AI+Africa Lead for the NLP-CoP: Vari Matimba
-
Do you see what I see? Insights from an inclusive approach to AI ethics governance.
-
New guide: key questions to ask before using GenAI for research on violence against women
-
RightsCon Recap – Assessing humanitarian AI: what M&E frameworks do humanitarians need in the face of emerging AI?