Recap: Using AI to Build a Localized and Participatory MEL System
The Sandbox group — part of the Natural Language Processing Community of Practice — organized a session on Thursday, June 13th, 2023, which explored an application of AI to create a participatory MEL system. The session featured Juan Carlos Muñoz Mora, professor of Development Economics and Chair of Policy and Development at Colombia’s Universidad EAFIT, who presented on his work leading the team in developing an AI-enabled MEL platform.
The platform in question is being developed by a team at EAFIT and supports monitoring, evaluation, and learning efforts for Safe and Sound Cities (S2Cities), a multi-country initiative founded by the Botnar Foundation and led by Global Infrastructure Basel (GIB). This initiative aims to assist selected cities worldwide in implementing youth-led participatory interventions to enhance the safety and well-being of young people in urban environments.
The EAFIT system draws together primary data from youth (blogs, audio, photos, video, social media) and secondary data (in the form of reports, work plans and other MEL inputs) from cities in the Philippines, Colombia, Ecuador and, Indonesia. It uses AI tools to help draw out conceptual dimensions of change according to the localization of program’s overall theory of change and its own learning framework (Evidence to Action) framework.
Through his presentation, Juan Carlos expanded on the challenges his team sought to overcome in creating an effective and participatory MEL system, along with the strategies they used to overcome these challenges.
An academic’s ideal is not always practical for local partners
Juan Carlos began his presentation by laying out the project’s ambitions and the roadblocks his team soon ran into.
When the EAFIT team first designed the MEL platform, they started with an idealistic framework. They created a theory of change with 85 indicators. Their partner NGOs liked the framework but did not have the time or resources to implement it. It was doomed to fall short of it’s ambitions.
Several problems surfaced, including:
- Commitment: Participants did send data, but often they did not send useful data – they treated the task as if they were doing homework in which they had very little interest.
- Capacity: The capacity of local and regional partners was a huge problem. They lacked both the resources and knowledge to sustain a robust MEL program.
- Participation: The team struggled to make the program participatory and involve youth in the design, production, implementation, and review of their program.
- Alignment: Another problem was fostering a common understanding of what constitutes quality evidence – this led to heterogeneity in what participants would submit.
Others in this field are no strangers to these challenges.
Becoming a Submarine
To address these issues, the team needed to change its vision for the project, and lean into practical constraints.
Juan Carlos outlined the team’s vision for a more flexible and participatory MEL system. The updated project aims to put MEL in the hands of young people by helping them document and report change on their own terms. Young people themselves do sensemaking along the way in an embedded process that transforms M&E from, as Juan Carlos puts it, “a heavy-duty, aggressive battleship into a low-key, invisible submarine” by enabling young people to report on their own meaningful changes and to use the capabilities of large language models and generative AI to support with data collection, analysis, and reporting.
In practice, they are transitioning to this submarine-like-state through three strategies.
First, they started working more closely with local partners. They transitioned from being a passive, traditional partner to being an active partner trying to understand local capacity and how they could better integrate the localization. One tactic they used to achieve this goal was to start using AI to foster ongoing partner participation and thus create more opportunities for engagement. Initially, they held workshops every two months, but they wanted more frequent involvement in order to make the reporting NGOs did useful for the NGOs themselves. They found that AI was an effective tool to help them reach this goal because it enabled them to deliver a flexible framework that partners could use to draw insights on demand. A key characteristic of the model they built – called Emma – was that the model was completely embedded into their theory of change, a quality that shaped the model’s insights according to the objectives of the overarching program. Generally, the EAFIT team got closer to local partners by creating more touchpoints and making the data useful to those who provided it.
Second, they doubled down on the idea of using new data collection methods as a way to encourage youth participation. They recognized that to create a participatory model, they needed to allow data to be more fluid, particularly since they were already getting various types of data from various sources because NGOs weren’t clear on what constitutes quality evidence. To address this, and to get youth to innovate, the team saw that they needed to embrace alternative ways of collecting data. They had to allow youth to “be themselves” and submit drawings, videos, pictures, or whatever else spoke to their experiences. The team made space in its system for diverse data and micronarratives.
Third, the team recognized that they needed to address the ethical and privacy challenges that arose through collecting primary data from youth. Names and other identifying information would often come through in the data, and these needed to be scrubbed before they were fed into the AI system. The team created a framework for pre-processing the data by removing sensitive information and transforming all data forms into text. This is a completely offline process. Then the data was translated into English. By the end of this process, the diverse and potentially compromising data was standardized into a format that could be safely integrated into a broader system.
An illustration of the full Emma system is included below. It outlines the process by which data is collected, anonymized, standardized, fed into the AI system, and then made available for local partners to use for their own purposes.
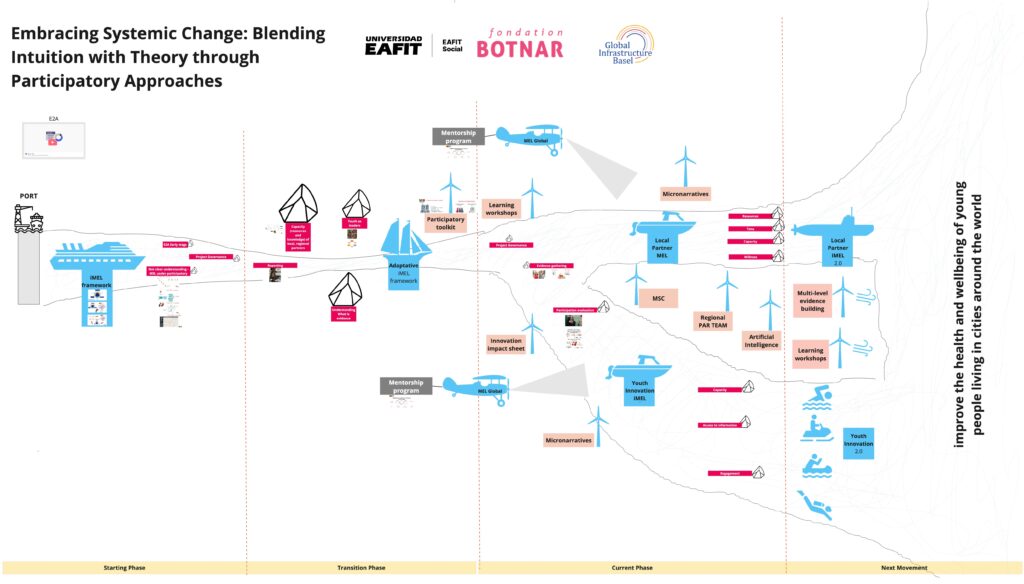
Key Learnings and Takeaways
Several insights arose through the presentation and Q&A that may be relevant for others interested in this approach.
Develop systems to enhance engagement and utility of data for reporting NGOs
- If the partners who are responsible for submitting data are disconnected from the data’s learning value, the quality of the data they provide will suffer, as will the overarching objective of creating a participatory MEL system.
- Commitment to the system can be increased by providing more frequent touchpoints with partners and designing these touchpoints to meet their needs.
- AI can be a powerful tool for delivering value to partners, as it offers an accessible way for them to obtain insights that support their own efforts. Furthermore, AI serves MEL needs by enhancing analytic capacity.
- AI models can better support program objectives by being embedded into the program’s theory of change. When AI systems are designed in this way, outputs are shaped according to the theory of change and can enhance understanding of data in terms of the programmatic vision.
Embrace flexible, diverse and innovative data collection methods
- Rather than require partners to conform to standardized data submission criteria, MEL systems may be better served by meeting partners where they are. By embracing innovative data collection methods, more voices can be incorporated, data can be enriched, and buy-in from partners can be enhanced.
- To incorporate flexible and diverse data collection methods, MEL system organizers will need to build an accompanying process for standardizing this data. Some of this process may need to be manual, but there are many tools available for automating the translation of various data into text.
- By being more flexible, MEL systems can help programs better live up to objectives such as being more participatory and empowering youth.
Address ethical and privacy concerns through a rigorous pre-processing framework
- Ethical and privacy concerns can be addressed through meticulous pre-processing. This process will likely need to be done manually so that a discerning human actor can sort through and apply complex ethical criteria when preparing raw data.
- Safeguarding privacy can be particularly challenging with working in a multilingual context. Though it may be easy to spot and remove identifying information when working in one’s own linguistic and cultural context, it can become much more difficult when working across these barriers. In these cases, it becomes important to work with local partners who have the requisite knowledge to maintain ethical and privacy standards.
Next Steps
- Participants at the session were interested in learning more about practicalities of Emma. The Sandbox is organizing a follow up live chat session with Juan Carlos in July to explore outstanding questions about Emma. More information is coming soon!
- Be sure to join the NLP-CoP if you’d like to stay connected and receive more information about events like this.
Learn more about how you can support the NLP-CoP and its working groups. If you are interested in sponsoring or supporting this work, please get in touch – we’d love to chat!
You might also like
-
We’re hiring: Join MTI as our new AI+Africa Lead for the NLP-CoP
-
Welcoming our new NLP-CoP Community Manager: Bárbara Paes
-
Join us for a special NLP-CoP members-only event with: Mirca Madianou, author of Technocolonialism
-
Humans in the Machine: the Impact of AI on workers – Learn More on February 6th