The US won’t be regulating AI climate impacts. Are there sustainable AI alternatives?
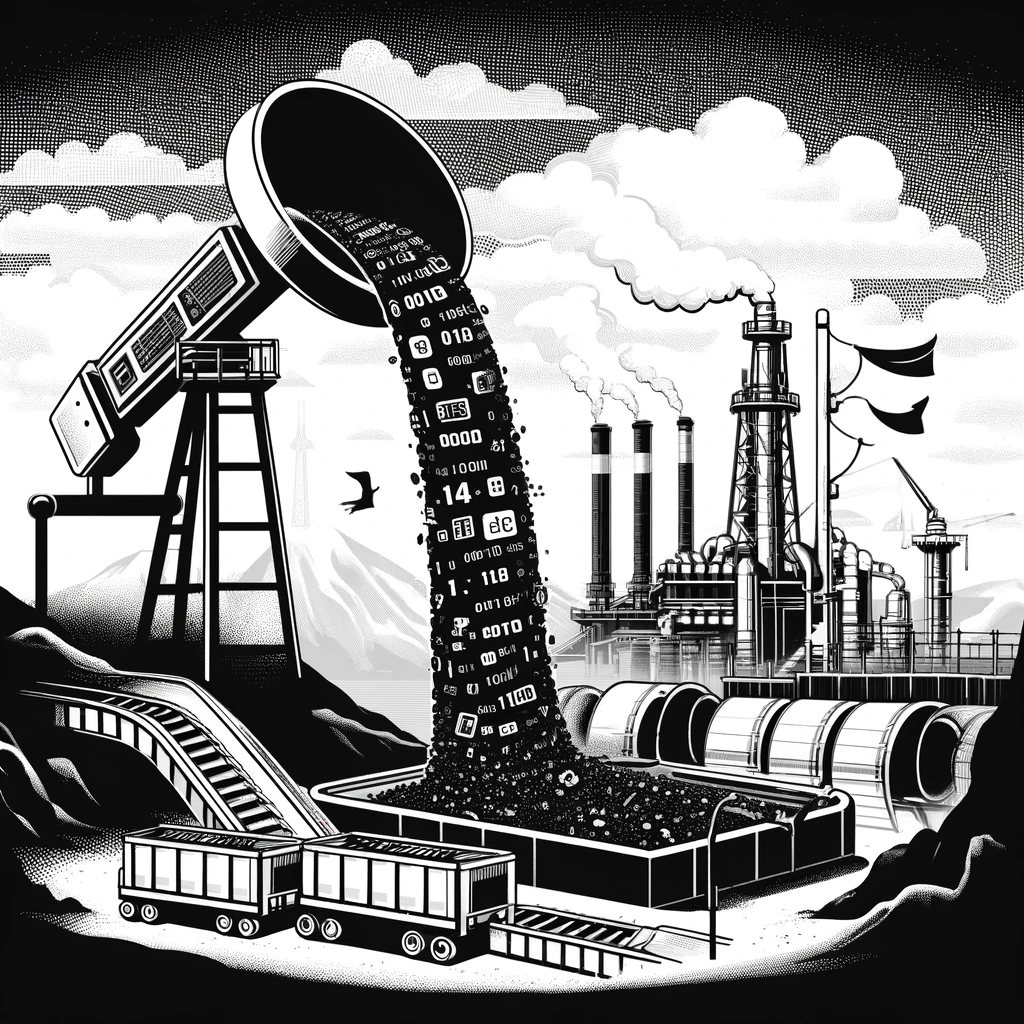
Like many, I’ve become much more aware of the intersection of climate and Artificial Intelligence (AI) over the past year. Last November at the European Evaluation Society’s virtual convening I spoke about ethical issues with Generative AI, including bias, equity, problematic labor chains, and climate. This September, I joined a small off-the-record convening on Climate Evaluation and Learning at Rockefeller’s Bellagio Center where AI was a key topic. At our workshop and keynote panel at the American Evaluation Association conference in October, we covered the topic of ethical AI, including climate opportunities and impacts.
With a new administration set to take over in the United States in January 2025, we can expect the US to retreat from any efforts to reign in dangerous levels of global warming. While AI and the data centers that allow it are not the biggest issues when it comes to climate, the tech industry is one to keep an eye on.
Over the summer I worked with Bhavin Chhaya to take a look into how advanced data analytics, predictive analytics and various types of AI are both supporting climate action and contributing to global warming and environmental degradation. Bhavin’s rapid research highlighted how AI is being used to support climate action in multiple ways, including:
- Setting prices, forecasting demand, and creating trading platforms for second hand markets (ThredUp | Fast Company)
- Using visual imaging techniques to accelerate the food inspection and reduce food waste in supply chains (Leanpath)
- Generating evidence to promote responsible business practices in the fishing industry (Global Fishing Watch)
- Enhancing environmental pollution monitoring and management (Simona et al), among others.
- Deepening understanding of the effectiveness of climate policy (Climate Policy Radar)
- Increasing the collective impact of the ecosystem of climate funders (Vibrant Data Labs)
At the same time, the research painted a concerning picture in terms of negative impacts of AI, including Generative AI, on the environment.
Energy and emissions
In terms of energy and emissions:
- Global emissions from cloud computing already range from 2.5% to 3.7% of total global greenhouse gas emissions, exceeding even those from commercial flights (2.4%).
- By 2030, the energy required for AI model training and data processing could consume up to 3.5% of global electricity.
- Major cloud providers like Meta, Amazon, Microsoft, and Google have seen their energy usage more than double between 2017 and 2021.
To meet the pledge to customers that their data and cloud services will be available anytime, anywhere, data centers are designed to be hyper-redundant. In some cases, only 6 to 12 percent of energy consumed is devoted to active computational processes. The remainder is allocated to cooling and maintaining chains upon chains of redundant fail-safes to prevent costly downtime. AI models are more or less energy intensive, depending on how they are designed and the tasks they are designed to perform.
Different data centers have varying carbon footprint, energy demand profiles on the grid and levels of efficiency. According to one study, the choice of deep neural network, data center, and processor can reduce the carbon footprint up to ~100-1000x. Overall, there is insufficient understanding of the range of factors that influence the efficiency of Large Language Models (LLMs) and their training. There is no mandatory reporting on training time, published performance results, cost–benefit analysis or environmental costs of Natural Language Processing models for comparison, making it difficult to track and monitor.
Water
The environmental impact of AI also includes water consumption.
- In 2022, Microsoft’s Iowa data centers consumed around 11.5 million gallons of water (6% of all the usage in the district) during GPT-4’s training period—which happened during a time of severe drought in the region.
- Google reported a 20% increase in water use for AI development in 2022, while Microsoft’s water consumption rose by 34% (according to annual sustainability reports).
- These operations consume the most water on hot days, precisely when human demand for water is highest, adding to stress on local water supplies.
While companies have made commitments to be water neutral or positive, the efficacy of water positive and water stewardship claims rests on assumptions, limited measurement frameworks, and geographical mismatches. For example, not all ‘water replenishment projects’ actually replenish water. These projects might include providing access to water, wastewater treatment capacity enhancement, irrigation efficiency, crop rotation, and wetland conservation. Hence, the notion of being ‘water positive’ is misleading. Water consumption is increasing and water is moving from local communities to BigTech, raising human rights and inequality concerns. In addition, replenishment project calculations are normally done over the project lifetime, whereas consumption is calculated yearly. In addition, water replenishment doesn’t always occur in the same geography where the water is depleted.
Microsoft’s own sustainability report states the limitations explicitly: “Replenishment is a nascent market with limited guidance on what it means, how to account for benefits, how to make credible claims, and how to ensure replenishment investments are having a significant impact in high-stressed basins. Furthermore, the supply of replenishment projects in many global markets is limited or non-existent.”
As Bhavin also noted, there’s an intangibility to AI that makes environmental footprint accounting nebulous. With cars, you can see the fumes. With AI, you can’t see the cloud-based servers being queried, or the chips rifling through their memory to complete the processing tasks asked of it (see here, here and here for more background).
Rules and regulations?
While we may have hoped that regulation of data centers’ energy and water use was on the horizon, the unfortunate election of Trump for a second term makes this extremely unlikely. The incoming administration instead will continue its push towards deregulation, people and planet be damned. In the four years of the first Trump administration, according to The Brookings Institute, 74 actions had been taken by the Trump administration to weaken environmental protection.
So what do we do? Are there alternatives?
Given all this, I’ve been wondering whether the sustainable, positive use of AI is even possible. If we care about climate and the environment, and we also want to use AI to improve climate action and climate learning, what do we do? We won’t be seeing AI or environmental regulation any time soon, and making individuals feel guilty about their use of AI is probably not the best way to go about it. Are there bigger picture, sustainable and structural ways that the social sector and MERL practitioners can consider the impacts of AI on climate and support climate friendly AI?
Cathy Richards, (one of the MERL Tech Initiative’s Core Collaborators), has been looking into the future of climate-friendly and ‘small’ AI for a while. We had a conversation in August (see below) where Cathy laid out what it is, its possibilities, and the challenges we face. In light of sobering election results, we felt it was important to share what Cathy has been thinking about.
How did you first begin exploring this?
It actually all started in the NLP-CoP! Someone shared a series of resources in the AI+Africa WG last year – in one case, a project combined AI and IoT to aid small-scale farmers, especially those with limited resources. In another case, scientists developed an LLM that aimed to address the need for models that are “efficient, accessible, and locally relevant, even amidst significant computing and data constraints”. Then late in 2023, I was selected to participate in the Green Web Fellowship and saw an increase in conversations around geospatial AI, and it hit me: In the process of trying to save the planet we’ll likely also cause it more harm.
That’s when I started to look more into more sustainable approaches to AI. An article on the need to rewild the internet really opened my mind to the possibility that things can be different. Earlier this year, Joana Varon, Sasha Costanza-Chock, and Timnit Gebru also did a great job of outlining a series of recommendations for shifting AI development away from big tech. The local first movement, which I’m a huge fan of, served as inspiration for this as well. I was able to explore the more creative side of things by visualizing this concept during a Deep Dive Week while at my previous job at The Engine Room.
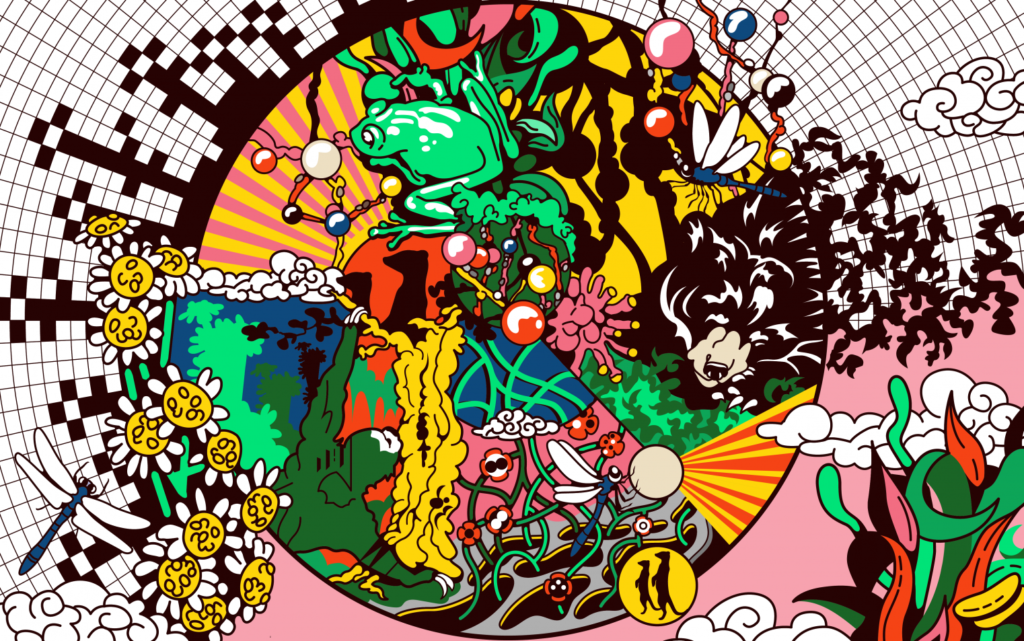
What are the key features of climate-conscious, small AI?
From my research so far, small, climate-conscious AI would be:
- Federated/Decentralized. It should move away from centralized, resource-intensive Big Tech systems that are ultimately susceptible to failure.
- Low resource: Climate-conscious AI should be able to function using minimal resources both from a data and computing power perspective. By prioritizing low-complexity models and smaller datasets that focus on specific, local needs, we not only reduce the energy footprint but also mitigate biases often introduced in massive, scraped datasets.
- Transparent: It should be transparent, especially regarding its carbon and energy usage. Incorporating climate benchmarks into repositories allows users to understand the ecological impact of the models they’re using.
- Work with nature: Projects like Solar Protocol showcase how AI systems can operate according to environmental dynamics, running only when renewable resources are available. This design promotes an energy-centered rather than resource-centered AI, mimicking natural intelligence by allowing environmental conditions to inform usage patterns. Similarly, incorporating “sleep” or “hibernation” modes based on actual demand rather than peak capacity—like the way electric grids are often built—would prevent unnecessary energy consumption.
- Contextual: It should respect cultural diversity and local relevance, reducing the need for massive datasets in favor of smaller, culturally contextual datasets. Initiatives like those of Indigenous AI and decolonial AI push us towards a world where…As Ameera Kawash stated: “I believe we should start from very small, local instances. For example, I am working to involve real-world cultural institutions in the creation of datasets, thereby developing highly curated and customized models to train AI without scraping the internet. This approach helps resist the exploitation that typically underpins the making and training of these technologies, which is also where most biases are introduced.“
- Intentional: We need to ask ourselves if we really need AI for the task at hand. As Sasha Luccioni, climate lead at Hugging Face stated, do we really need AI to navigate from point A to point B?
Where are the opportunities?
Unlike conventional AI, which often requires extensive, centralized data centers with high energy consumption, and constant internet access, small AI models—like those used in edge computing can operate efficiently on local devices with minimal power requirements. By lowering the need for continuous internet connectivity and reducing reliance on large data centers, these models make it possible to use this technology not only in low resource contexts but in low demand ways.
What are the challenges?
One of the main challenges we face is a lack of financial interest from major tech companies. The current models and business structures make them money. Varon and the other authors go into this in the policy briefing, reminding us that there’s no government oversight for the current extractive systems.
Then of course, we do need to think about capacity building – making sure that communities and organizations have the skills and resources to adopt and support these alternatives effectively.
We also have to make the world aware that these options can also exist.
How do we get there?
Rather than accepting that the cards are dealt and we have to use Big AI systems, Foundations and other donors could invest in green, small AI to see if it’s possible to use AI to tackle climate challenges without contributing to them.
Engage and learn more about the intersections of Climate, MERL + AI
Cathy will be starting up a Working Group on this topic within the NLP-CoP. Look for more information coming soon through normal NLP-CoP channels. If you’re not yet a member of the NLP-CoP, you can join up here and choose to be a part of this (and other) working groups.
You might also like
-
We’re hiring: Join MTI as our new AI+Africa Lead for the NLP-CoP
-
Welcoming our new NLP-CoP Community Manager: Bárbara Paes
-
Join us for a special NLP-CoP members-only event with: Mirca Madianou, author of Technocolonialism
-
Humans in the Machine: the Impact of AI on workers – Learn More on February 6th