Meeting Recap: Using NLP for Qualitative MERL in Africa
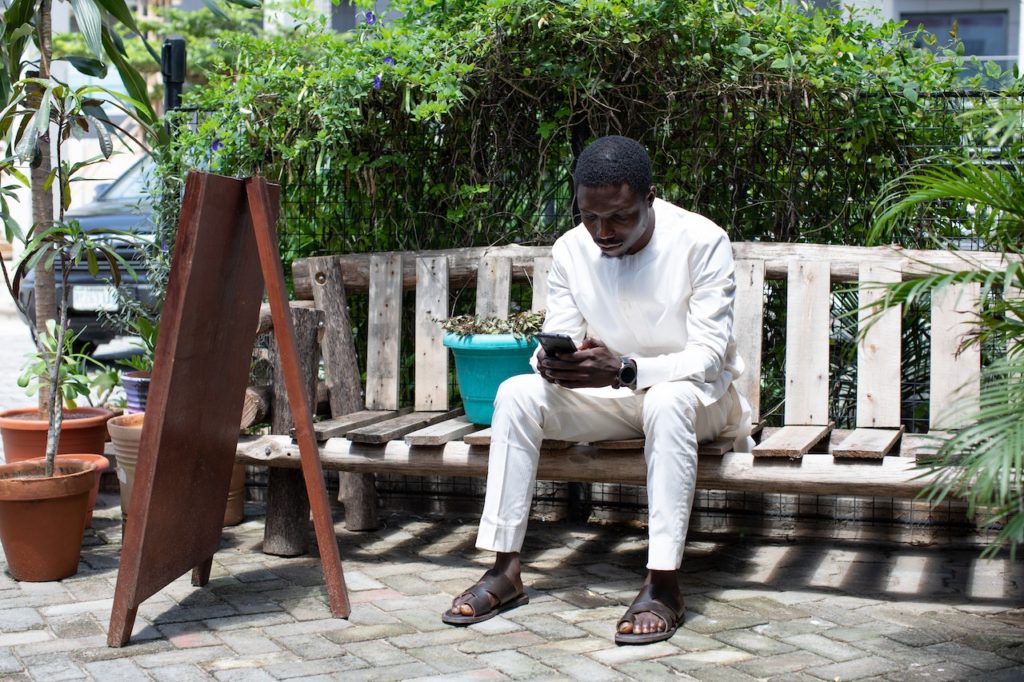
On the 27th of June, 2023, MERL Tech’s Natural Language Processing Community of Practice (NLP-CoP) and the South Africa Monitoring & Evaluation Association (SAMEA)’s Tech-Enabled MERL CoP co-hosted an open meeting on Using NLP for Qualitative MERL in African Contexts with 59 participants in attendance.
Our speakers were Korstiaan Wapenaar from Genesis Analytics, South Africa; Jerusha Govender from Data Innovators, South Africa; and Mmoloki Sello from Botswana Family & Welfare Association, Botswana. Topics discussed ranged from the step-by-step technical details of how Large Language Models (LLMs) work, to the landscape and use cases of NLP in Africa, the interests of different stakeholders, the questions around bias and cultural norms to be considered, and the opportunities for MERL.
The event was attended by esteemed MERL professionals and consultants, researchers and academics, as well as business professionals and other interested parties. The diverse audience hailed from a broad range of locations including Uganda, Nigeria, Ghana, Edinburgh, London, Tanzania, Kalamazoo, New Orleans, New York, Minneapolis, and South Africa. Professionals from key organizations such as NIRAS, GIZ, IRI, Save the Children, UNHCR & UNICEF were also present.
Watch the recording and access the meeting slides here.
Background on LLMs, main drawbacks, and opportunities for increased value and efficiency
Korstiaan Wapenaar from Genesis Analytics, South Africa, kicked off the discussion with a talk on LLMs as a step-change in NLP. He offered background on how LLMs are a novel feature discovered in 2017 that has greatly expanded the capabilities of Artificial Intelligence (AI), and explained that recent computational improvements have enabled the current emergence of LLMs. A profound extension to traditional NLP is their ability to perform multiple tasks as instructed by the user. Korstiaan proceeded to give an overview of how LLMs actually work based on prediction of the next word, and how this predictive power is enhanced by extensive training on “large” language datasets and models. Wapenaar shared a useful breakdown of what LLMs are good and bad at.
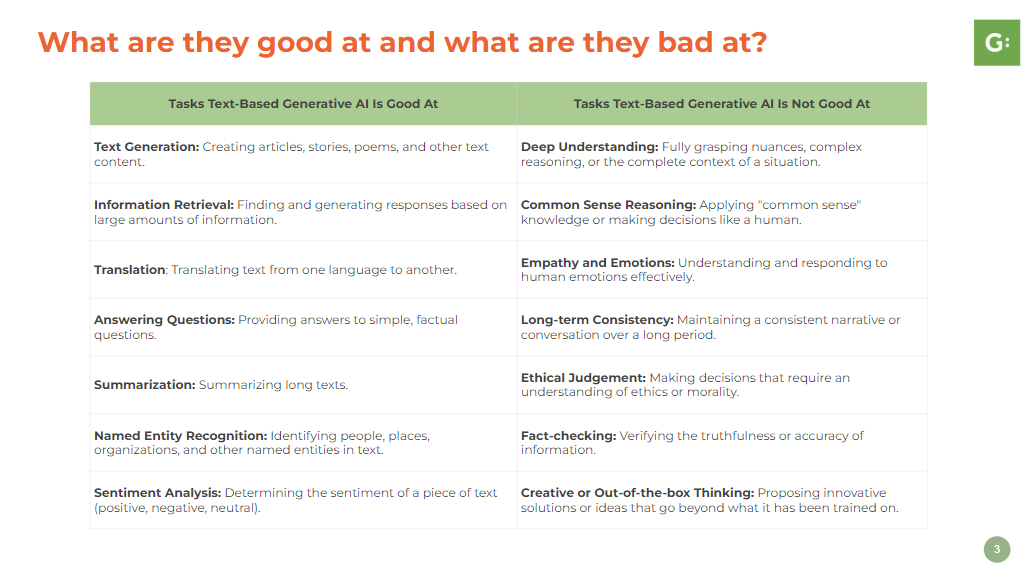
He highlighted an additional challenge: “AI hallucinations” (convincing yet inaccurate responses received from AI chatbots). The implication of this for analysis is that researchers and other users have to be very careful and well-versed on the content and related facts to which they are applying LLMs. Open-source alternatives are becoming more prominent, he noted, and this may provide opportunities for improving LLMs. Korstiaan closed off by imploring analysts to think more deeply about how they can provide value and differentiate themselves.
AI use cases in Africa, limitations and risks
Jerusha Govender from Data Innovators, South Africa, took the discussion further by sharing some potential use cases for MERL including time-saving, analysis, cost-reducing enhancements to the broad scope of qualitative MERL processes, for example, lengthy document reviews and other heavy content-based interventions. “Content and text engagement” is often relied upon but critiqued for its inherent biases and non-exhaustive nature, but the use of AI makes it a potentially credible method of evaluation in the near future. The generation of foresight is also made possible due to the broad array of information and historic information available for summarizing.
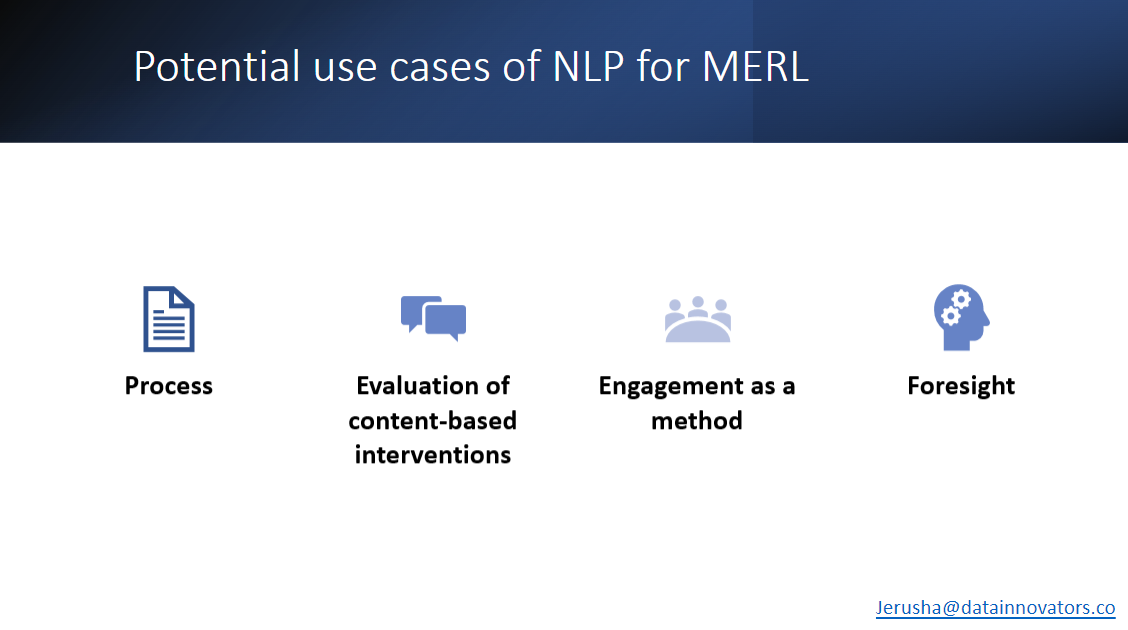
Some of the use cases that Jerusha shared include
- an open-source M&E system called Monic that integrates ChatGPT to summarize closed-ended qualitative responses to questionnaires
- a text analytics API integration called Lexalytics that hosts a vast array of machine learning, sentiment analysis, and AI research project outputs for knowledge sharing
She mentioned grantmakers like Lacuna Fund, who are supporting the development of inclusive and equitable datasets in low & middle-income contexts, including projects by African researchers across many domains. She cited a project funded to develop datasets of the Hausa, Ibgo, and Yoruba languages from Nigeria and increase their availability for machine learning models and NLP tasks such as sentiment analysis, emotion analysis, hate speech detection, and fake news detection. Jerusha highlighted that the currently available AI platforms do come with risks because they are not perfect, and they may be perceived to be fast replacing evaluator tasks, which is a limiting factor to remain cognizant of.
Ethical concerns: Bias, transparency, and cultural considerations.
Mmoloki Sello from Botswana Family Welfare Association closed off the panel presentations with a talk on the ethical considerations of bias, transparency, and cultural nuances. Sello called for the transformation of AI’s “black box” into an interpretable “white box”, and he advocated for the responsible and inclusive development of AI for the greater good. By juxtaposing the accountability of AI against human accountability to God, laws, and fellow humans, he illustrated the necessity of a governance framework and attention to AI ethics, with careful oversight on some of the risks that Jerusha had highlighted, as well as those listed below:
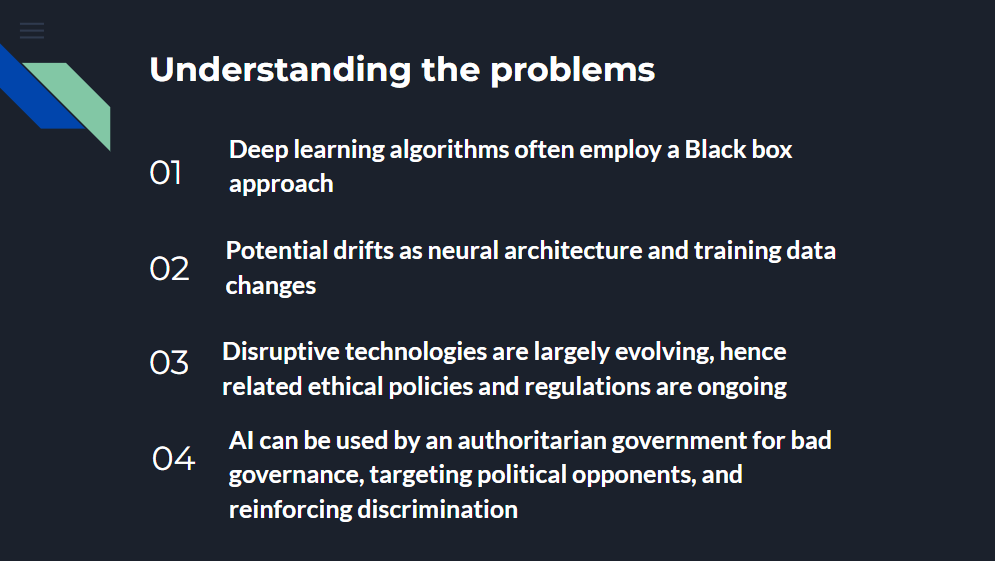
Reflecting on OECD AI Principle 15 – accountability and advocacy for controlled experimentation and transparent environments for AI testing and innovation – Sello noted the absence of African perspectives in the discourse. This is further hampered by the limited comprehensive data protection and privacy legislation in 38 of the 55 African Union (AU) member states. That said, Mauritius stands as a pioneering African nation with its AI strategy and National AI Council.
Sello highlighted the importance of inclusive AI ethics frameworks that address diversity, biases, and contextual variations by incorporating different perspectives in the pursuit of technical solutions that are designed effectively for their audience. In addition to increased knowledge of what constitutes bias, it was emphasized that Africa safeguard its data privacy and protection against foreign exploitation by incorporating strong data protection regulations, enhancing capacity and expertise, conducting risk assessments, raising public awareness, improving algorithm transparency, and emphasizing collective rights alongside individual rights in African contexts.
With privacy being a major concern, the audience frequently raised questions on prompt engineering and the accuracy of the responses obtained.
Prompt engineering best practices
A common theme amongst the audience was an interest in how to structure prompts that work well and avoid over summarizing and losing accuracy in LLMs, and how to incorporate the subtle differences that produce different and better responses from LLMs. Aligned to this, was further interest on how frequently LLMs produce “correct” responses to prompts. There exist several resources where experts have attempted to summarize best prompting practices such as this article by Topbots.com. The continuous re-emergence of this topic has warranted efforts by the working group to provide an opportunity for further discussion and practical engagement which members can look forward to on August 30. (Join the CoP if you’d like to attend!)
Privacy concerns and locally run LLMs as a solution
Privacy and data protection were also common themes interrogated regarding the use of models such as ChatGPT, especially since MERL data often includes identifying information. It was noted that this concern is driving innovation in LLMs that are “privately operated” which is becoming increasingly common. Privately run LLMs such as H20, offer an option to ensure data protection by allowing you to train the models on your own data, however, their lack of connection to “larger” models may mean a compromise in their accuracy, and this is yet to be explored further.
ChatGPT also provides opt-out settings for using your inputs for training. A drawback of this setting is that you no longer have the chat “history” function so it is difficult to develop insights in the “conversational” format that allows you to revise prompts and further lines of inquiry. ChatGPT recently released new features to help manage privacy that can be read about here.
The context in which these conversations are critical is with direct reference to the African continent where only 12 of over 200 languages are fully represented in platforms such as Google translate. Access to the digital landscape and all related opportunities is significantly hampered when you do not speak a well-resourced language, which is a concern for many under-served and under-represented communities. The presence of platforms such as the NLP in Africa Community of Practice offers an opportunity to center the voices that are yet to be heard and advance solutions that take into account their nuanced livelihoods.
“African ethicists and MERL practitioners are in a good position to develop Africa’s ethical frameworks because of their valuable awareness of the African societal norms and values.” Mmoloki Sello, 2023
If you’ve read this far and would like to continue being a part of these conversations either as an observer, contributor, or partner, you can find information on how to join the NLP Community of Practice here.
Leave a Reply
You might also like
-
What’s happening with GenAI Ethics and Governance?
-
Join the AI and African Evaluation Working Group Meet ‘n’ Mix Session on May 7!
-
Hands on with GenAI: predictions and observations from The MERL Tech Initiative and Oxford Policy Management’s ICT4D Training Day
-
When Might We Use AI for Evaluation Purposes? A discussion with New Directions for Evaluation (NDE) authors
This article is so helpful, thank you.