What’s next for Emerging AI in Evaluation? Takeaways from the 2023 AEA Conference
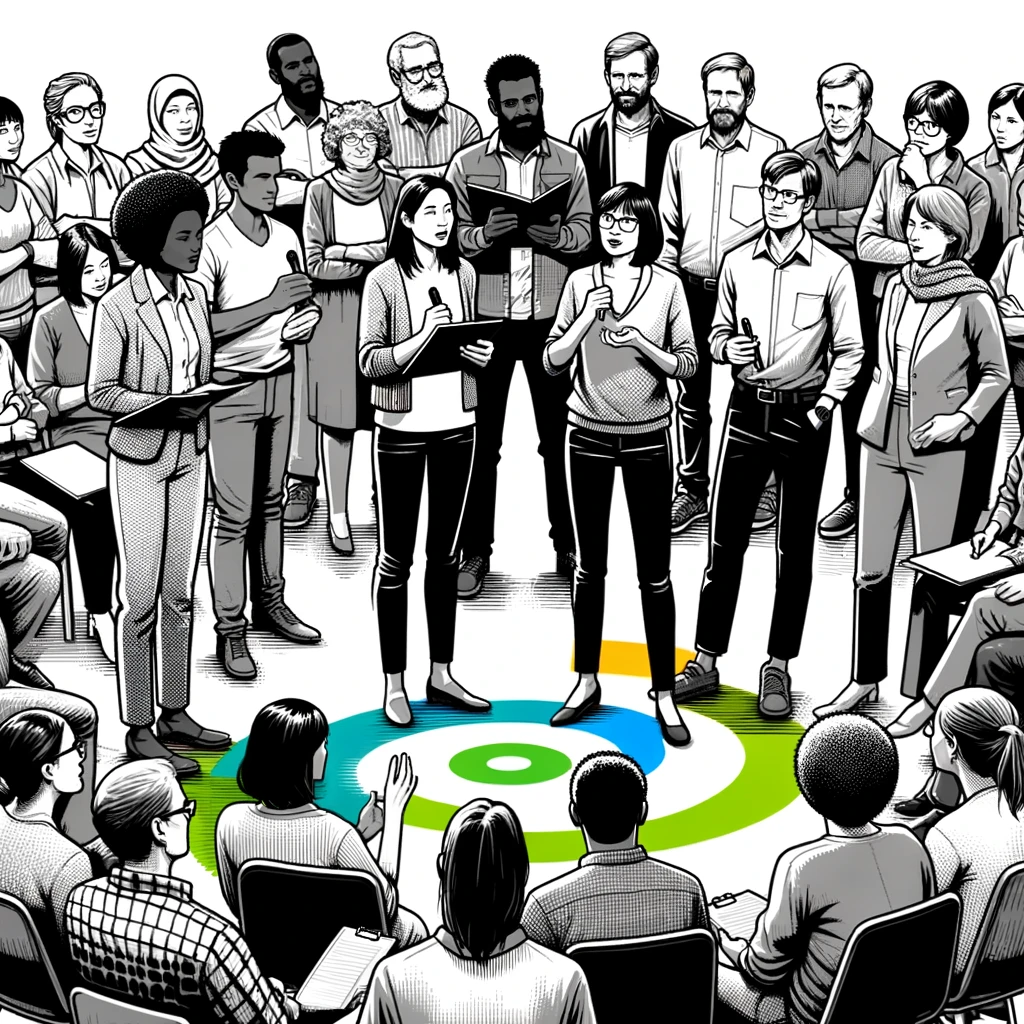
This post is co-authored by Zach Tilton (tech-enabled evaluation consultant and PhD candidate at the University of Western Michigan) and Linda Raftree (MERL Tech founder and Natural Language Processing Community of Practice lead).
This year’s American Evaluation Association (AEA) Conference was bursting with interest in emerging Artificial Intelligence (AI). As two people following the trajectory of “MERL Tech” (tech-enabled monitoring, evaluation, research and learning) over the past decade, we are both excited by this and a bit daunted by the amount of change that natural language processing (NLP) and generative AI tools like ChatGPT will bring to the evaluation space. Like us, our fellow conference goers seemed both energized and fearful of these advances in AI. Read on for some of our key takeaways from the conference.
Our observations
Demand for guidance on AI-enabled evaluation at the AEA was high.
There were 18 presentations (many grouped together in a single session) on AI, machine learning, and big data. Sessions with ChatGPT in the title were standing—or sitting on the floor—room only, many with attendees filling the doorways. Sessions with “big data” or “machine learning” in the title received less attention (perhaps a “branding” issue?). Sessions varied in specificity, practical experience, and quality, with some questionable insights being offered to attendees including “You can tell ChatGPT to write an evaluation for you” (spoiler, you can’t—nor should you even if you could!).
Concerns about AI-enabled evaluation were evident.
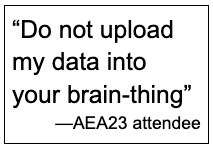
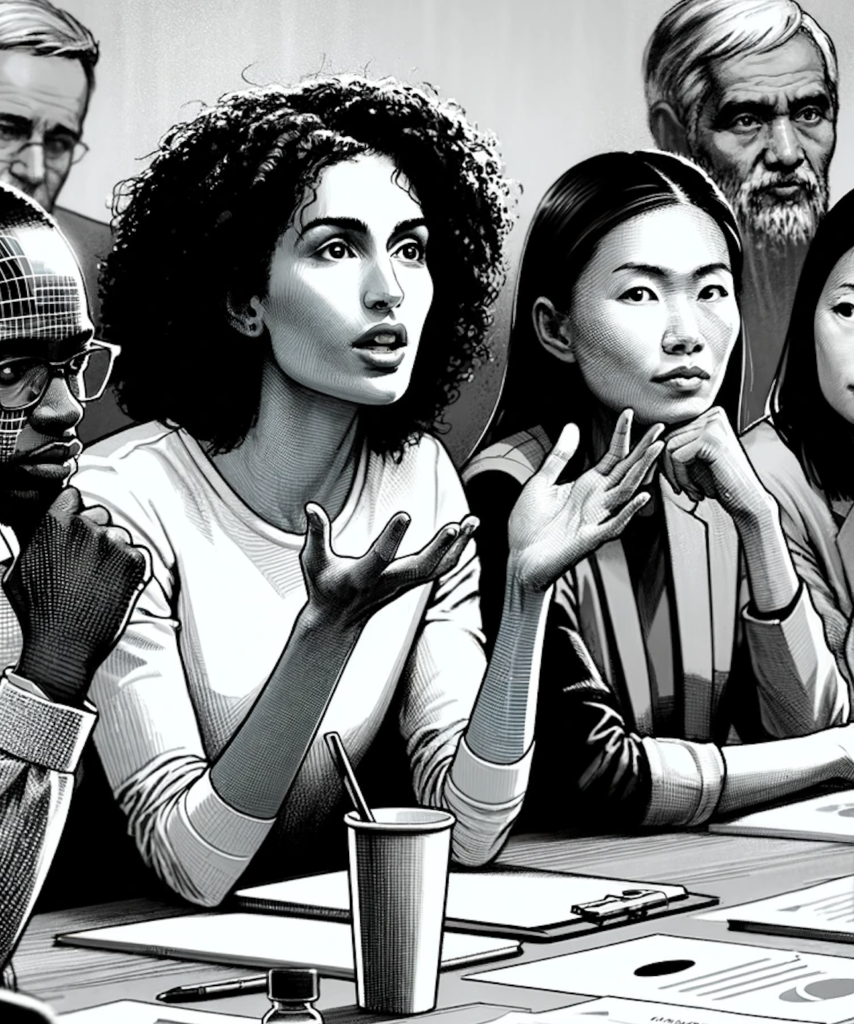
The questions from audience members were just as, if not more, interesting as the presentations themselves. If audience questions offer a window into the values and concerns of our field, an unrepresentative sample suggests many are concerned about data privacy and how AI technology will change our relationship with the craft of evaluation.
During one of many rich side conversations, a colleague working in the area of participation, inclusion and social change asked “What does it mean if we no longer ‘swim’ in the data?” As evaluators and researchers we live it, breathe it, dream it, and ponder it endlessly. We feel a kind of emotion or connection with data when we are “in it.” Do we want to hand that over to a machine to spit out some conclusions?
This concern chimes with an observation made by AEA23 presenter Thomas Schwandt more than 20 years ago that, “The more that teachers, counselors, administrators, social workers, and other kinds of practitioners look to outside experts to tell them what has value, the more these practitioners become alienated from the evaluative aspects of their practices” (2002, p. 3). Could it be that the integration of AI context-aware reasoning agents into our evaluation practice will show us the kind of alienation to which we may have inadvertently subjected our past clients?
We don’t really know yet what emerging AI can and can’t (or shouldn’t!) do for evaluation.
Participants at the AEA were looking for guidance on what these new kinds of AI are good for, where we might see gains from their use, where and when we shouldn’t use them, and what kinds of specific AI applications we might want to develop for evaluation. MERL Tech’s Natural Language Processing Community of Practice (NLP-CoP) has been looking at these issues since early 2023, yet we’re still scratching the surface, perhaps in part because some of the more popular, accessible applications (ChatGPT, Bard, Claude, etc.) have only been available to the public for one year.
One common finding in casual experimentation with emerging AI has been that it’s often not good at the tasks one would expect it to be good at. Researchers at Harvard and Boston Consulting Group (BCG) call this AI’s “jagged technological frontier” – certain tasks are easily done by AI, while other seemingly similar tasks are outside of AI’s capability. Their September 2023 paper describes an experiment conducted with 758 BCG consultants. Researchers gave consultants 18 realistic tasks considered within the frontier of AI capabilities and found that consultants using AI completed 12.2% more tasks on average, completed tasks 25.1% more quickly, and produced over 40% higher quality results as compared to the control group. While consultants across the board benefited from having AI augmentation, “those below the average performance threshold increas[ed] by 43% and those above increas[ed] by 17% compared to their own [baseline] scores.” For tasks considered outside of the AI frontier, consultants using AI were 19 percentage points less likely to produce correct solutions compared to those without AI for certain tasks that were deemed “outside the frontier.” (Similar studies have also found increases in productivity for specific tasks, and some gig workers have said that AI helps them do their work much more quickly).
These findings have interesting implications for the application of emerging AI to evaluation. One goal of the NLP-CoP is to map out this jagged frontier specifically for MERL. We can also consider these findings in terms of evaluation competencies, leapfrogging, and equity aspects. What skills will be needed for the future? How might AI enable MERL team members who show lower performance levels to achieve higher quality results? Is emerging AI just another tool – like a calculator or a dump truck – that will allow the need for a range of technical or computing skills to be bypassed? Some data science experts, including Pete York who presented at a session on addressing bias and big data and Paul Jasper who shared a demo where he asked ChatGPT to write code, suggest that the need for evaluators to learn R or Python, will go away. Critical thinking and theory, however, will remain vital in order to instruct and prompt AI towards specific outputs.
Some emerging conclusions
GenAI is more than vaporware.
Though there is no shortage of hype, noise, and thread bois gushing over generative AI, our sense is Gen AI is less ephemeral than Web3 and blockchain, though that’s not saying much (shout-out to our friends still working on distributed ledgers!). This likely means we can’t ignore this potentially disruptive technology. In fact, for many years now MERL Tech’s oracle, Michael Bamberger, has been saying there is a widening gap between evaluators and data scientists (those who have been traditionally working with machine learning and AI) and that the field of evaluation is putting itself at risk by not paying attention to developments with these emerging technologies.
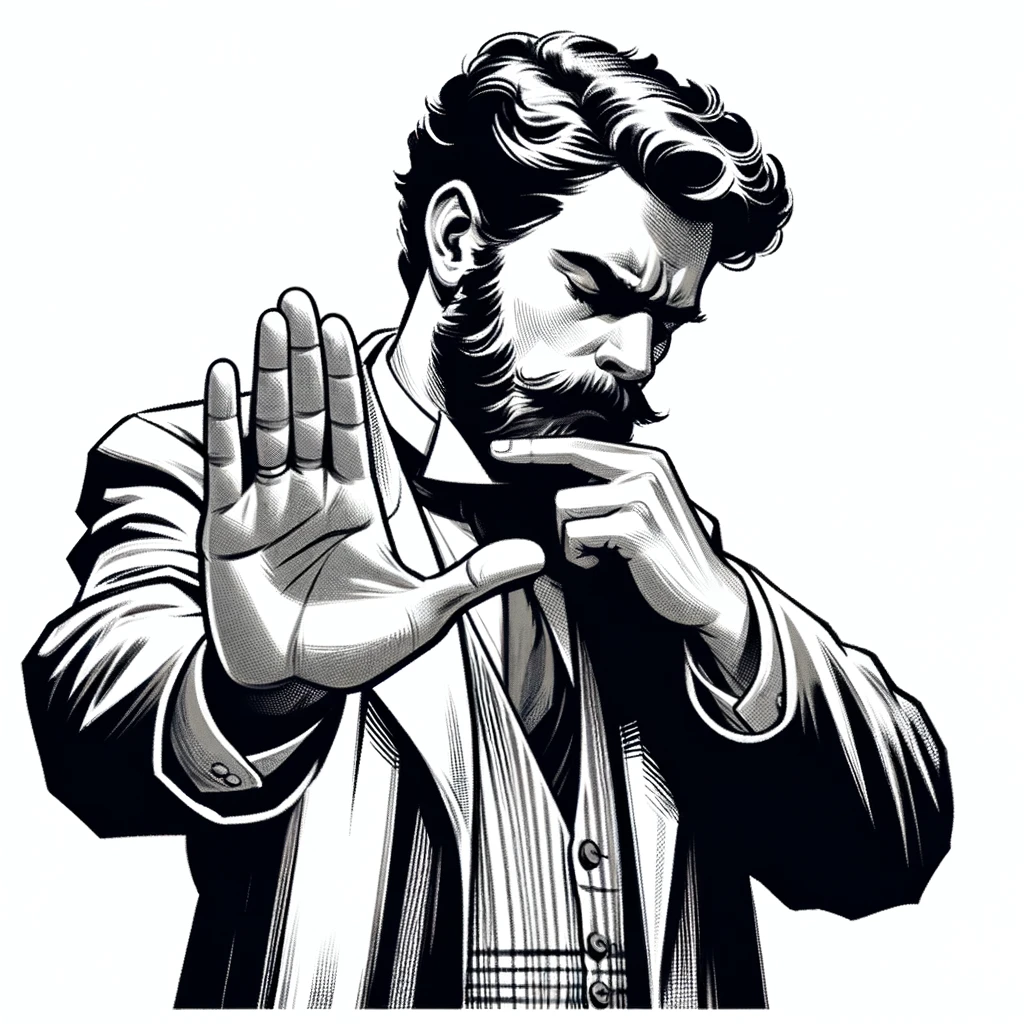
The risk is that if evaluators don’t engage with this tech or with those using this tech, despite long-standing efforts to recast evaluation as a value-based and morally informed culturally responsive social practice, it could relapse into an even stronger version of a so-called “value-neutral technocratic managerial enterprise” where unaccompanied computational statisticians and data scientists, who may be unaware of key issues and debates in evaluation practice, are asked to do more and more evaluation work as the current field of evaluation becomes less relevant. This topic came up at the very first MERL Tech conference in 2014, yet the idea seemed improbable at that point. In 2018, Rick Davies and Michael Bamberger debated it yet again. Now, in 2023, the issue is much closer at hand than we had imagined.
Many organizations will rush to build AI-enabled evaluation machines.
Our sense is that many evaluators are pining for guidance on AI in evaluation due to feeling behind the AI curve. Our experience working with organizations and evaluation units is that they feel this same pressure to get in front of and ride the AI wave and not be washed out by it. In The Evaluation Society, Peter Dahler-Larsen (who was in attendance at this year’s conference and entertained at least one philosophy of evaluation science discussion with us) writes about ‘evaluation machines’ as the “mandatory procedures for automated and detailed surveillance that give an overview of organizational activities by means of documentation and intense data concentration” (2012, p 176). The division of labor on large-scale evaluations and within evaluation systems already means a high-degree of alienation. With the prospect of further automation, evaluation in the age of AI may mean that even more.
The design of the evaluation machine is also separated from its actual operation. In other words, the “worker,” the “evaluation machine engineer, and the “evaluation machine operator” all perform different roles. Through the eyes of each of these role players, there is no total overview and total responsibility for work and its evaluation, although the results of the evaluation machine are said to stand for quality as such. This fixation on automation in the evaluation process may mean that although evaluation processes become optimized, this leads to only a semblance of gains in improving “micro-quality” while limiting society’s capacity to handle complex macro-oriented problems (p. 191).
We need to define research and upskilling agendas.
While some research and testing is being done on these newer forms of NLP and Gen AI in evaluation, (see the ICRC’s research and the World Bank’s IEG’s experiments, for example) the sector needs to do more testing and documentation on responsible application of emerging AI for various kinds of evaluation processes and contexts. The Fall issue of New Directions for Evaluation (NDE) (available for free to AEA Members) offers a great overview of these themes, and the NLP-CoP regularly shares and documents active learning, but ongoing, adaptive research is needed, especially considering how quickly the capabilities of AI change. A common expression over the last year has been that “ChatGPT3 is like a high school student, Chat GPT4 is like a masters level student.” So, what will GPT5 be able to do?
Research will be critical for helping the evaluation sector keep up with emerging AI approaches, identify areas of risk and potential for harm, and design the kinds of training and curricula that evaluators will need to use AI in their practice and to evaluate how others are using AI in their programs and how AI is affecting wider society at multiple levels.
So, what next?
Work now to future-proof your and our evaluation practice.
By no means are we saying that all evaluators should uncritically adopt AI tools and work at the frontier of tech-enabled evaluation. We do believe that evaluators should consider how AI and the fourth industrial revolution may alter their evaluation portfolio and the broader evaluation landscape. Evaluators may need to consider their unique value-add in an age of AI. What does human intelligence have to offer in evaluation that artificial intelligence can’t. In the face of this gap between evaluation science and data science, maybe evaluators should revisit and deepen the quiver of evaluation specific methodologies and consider how new technologies can strengthen and support the logic of evaluation. Finally, we should consider what the field and logic of evaluation have to contribute to emerging technologies, such as benchmarking in AI.
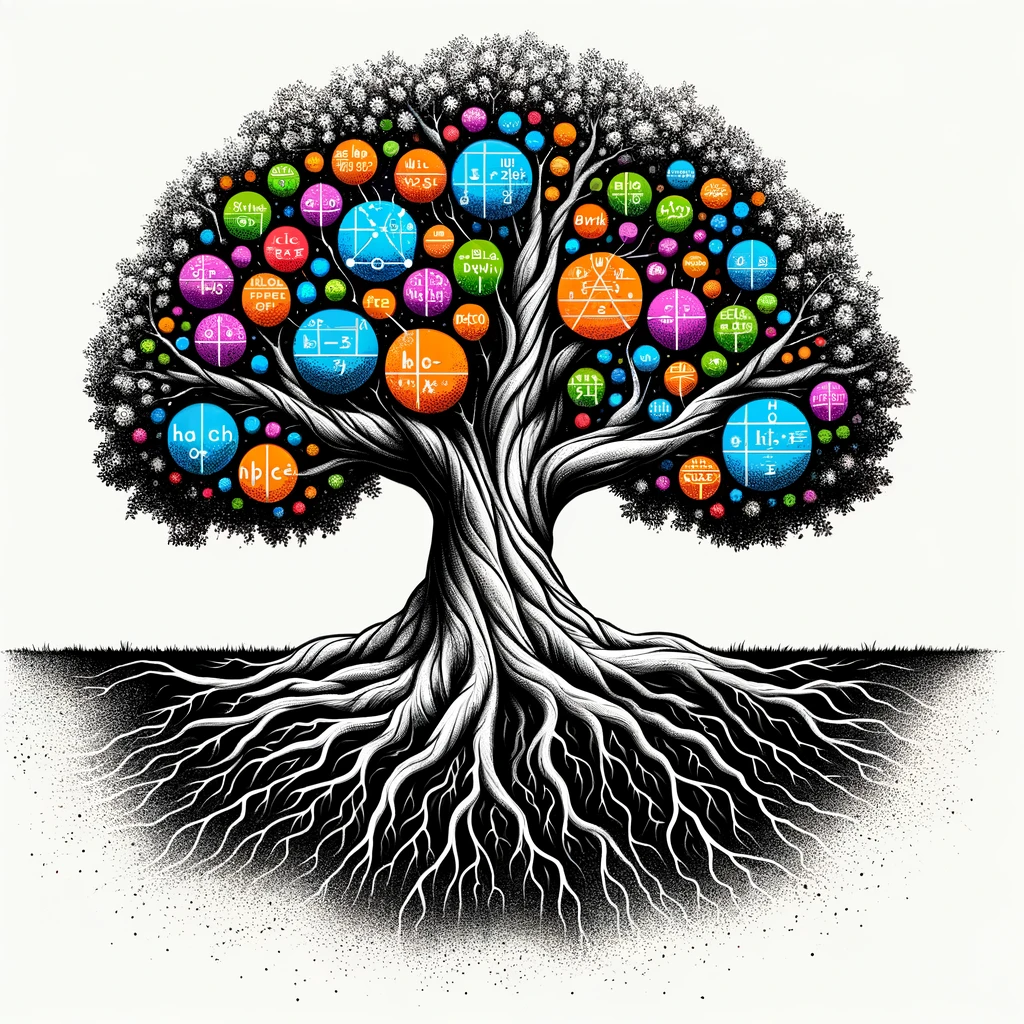
Avoid “theory free” AI-enabled evaluation.
Writing about the role of substantive or program theory in evaluation, Michael Scriven once claimed that explanatory theory is “a luxury for the evaluator.” He expounded that “One does not need to know anything at all about electronics to evaluate electronic typewriters, even formatively, and having such knowledge often adversely affects summative evaluation.” (1991, p. 360).
While this anachronistic example is somewhat true for consumerist notions of evaluation (especially if we think about our very scientific “vibe-checks” between the outputs of different LLM chatbots such as ChatGPT, Claude, or Bard) when it comes to the prospect of integrating this technology into evaluation and evaluative reasoning, we need to demand greater transparency in how this technology operates. In other words, evaluators don’t need to turn into computer scientists, but we do need a fundamental theory or understanding of how these technologies function so we don’t overextend AI’s role in our assisted sensemaking practice. The refrain at various sessions was to “Interrogate the technology, interrogate the data.” We can’t interrogate the technology when we don’t have a fundamental understanding of what the technology is or how it works. Evaluators need to get up to speed, and quickly!
Move forward on research, testing and upskilling.
The evaluation field as a whole needs to learn more about the low risk, high gain ways we can use emerging AI tools – where results are useful and valid and the potential for inaccuracies and harm are minimal. A non-exhaustive set of questions we might begin with includes:
- What does the ‘jagged frontier’ look like for emerging AI in evaluation? Can we achieve the same or better levels of efficiency or quality for certain tasks or processes when we use AI? Which ones? How could we measure, document, and share this information with the wider evaluation community?
- Where is automation possible and desired? Can emerging AI support high-level analysis tasks? How far can AI models go to create evaluative judgments? How far do we want it to go? Where is automation a bad idea? Where and how do humans remain in the loop? How can humans and AI work together in ways that align with institutional or sector-level values?
- Where do current AI tools and models offer reliable results? How could large language models be improved so that they are more consistently accurate and useful for MERL-related tasks? What level of error are we comfortable with for which kinds of processes, and who decides? What about accuracy and utility for non-English languages and cultures? How should various aspects of inclusion be addressed? Where does this overlap with data privacy aspects?
- What does meaningful participation and accountability look like when using NLP for MERL? What meaningful ways can communities and evaluands be involved when AI is being used? How can we do this practically? Is it possible to get meaningful, informed and active consent when AI is involved? How?
- Can emerging AI fill a skills or experience gap? Could AI tools enable young evaluators to more equitably access opportunities in the professional evaluation space? What AI-related skills do experienced professionals, institutional decision-makers, and evaluation commissioners need to have?
Opportunities abound for engaging with all of the above!
Linda will be speaking on “A Just Transition: What does it mean for AI and Evaluation?” at the European Evaluation Society’s Autumn Online Event on November 16. The theme of the African M&E Association’s conference in Kigali (March 18-22, 2024) is “Technology and Innovation in Evaluation Practice in Africa: The Last Nail on the Coffin of Participatory Approaches?” The 12th ICT4D Conference happens that same week in Accra, with a strong “Innovative Data for Impact track.” The AEA will have ongoing discussions as we move into the 2024 conference in Portland. Online discussions are happening via the NLP-CoP, EvalPartner’s Peregrine Discussion Group, and others. We encourage you to get involved where you can!
-
Pingback: What’s next for Emerging AI in Evaluation? Takeaways from the 2023 AEA Conference by Zach Tilton and Linda Raftree – AEA365
You might also like
-
What’s happening with GenAI Ethics and Governance?
-
Join the AI and African Evaluation Working Group Meet ‘n’ Mix Session on May 7!
-
Hands on with GenAI: predictions and observations from The MERL Tech Initiative and Oxford Policy Management’s ICT4D Training Day
-
When Might We Use AI for Evaluation Purposes? A discussion with New Directions for Evaluation (NDE) authors